Intelligent Wireless Networks: Challenges and Future Research Topics
- Published: 21 October 2021
- Volume 30 , article number 18 , ( 2022 )

Cite this article
- Murad Abusubaih ORCID: orcid.org/0000-0002-6948-1311 1
965 Accesses
8 Citations
Explore all metrics
Recently, artificial intelligence (AI) has become a primary tool of serving science and humanity in all fields. This is due to the significant development in computing. The use of AI and machine learning (ML) has extended to wireless networks that are constantly evolving. This enables better operation and management of networks, through algorithms that learn and utilize available data and measurements to optimize network performance. This article provides a detailed review on cognitive, self-organized, and Software-defined networks. We discuss ML concepts and put emphasis on how ML can contribute to the development of optimal management solutions of wireless networks. A focus is put on discussion and analysis of recent research trends and challenges that remain open and require further research and exploration.
This is a preview of subscription content, log in via an institution to check access.
Access this article
Subscribe and save.
- Get 10 units per month
- Download Article/Chapter or eBook
- 1 Unit = 1 Article or 1 Chapter
- Cancel anytime
Price includes VAT (Russian Federation)
Instant access to the full article PDF.
Rent this article via DeepDyve
Institutional subscriptions
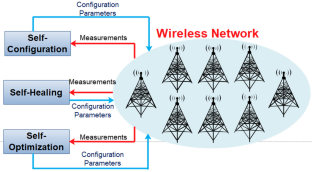
Similar content being viewed by others
Next Generation Wireless Communication: Facilitated by Machine Learning
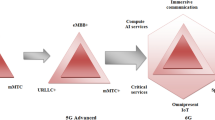
A Comprehensive Review on Machine Learning-based Approaches for Next Generation Wireless Network
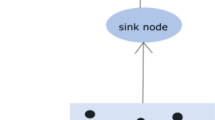
Machine Learning in Wireless Sensor Networks
Explore related subjects.
- Artificial Intelligence
Zhao, Y., Li, Y., Zhang, X., Geng, G., Zhang, W., Sun, Y.: A survey of networking applications applying the software defined networking concept based on machine learning. IEEE Access 7 , 95397–95417 (2019)
Google Scholar
Elsayed, M., Erol-Kantarci, M.: AI-enabled future wireless networks: challenges, opportunities, and open issues. IEEE Veh. Technol. Mag. 14 (3), 70–77 (2019)
Mao, Q., Hu, F., Hao, Q.: Deep learning for intelligent wireless networks: a comprehensive survey. IEEE Commun. Surv. Tutor. 20 (4), 2595–2621 (2018)
Zhang, C., Patras, P., Haddadi, H.: Deep learning in mobile and wireless networking: a survey. IEEE Commun. Surv. Tutor. 21 (3), 2224–2287 (2019)
Hämäläinen, S., Sanneck, H., Sartori, C., Self-Organising, L.T.E.: Networks (SON): Network Management Automation for Operational Efficiency. Wiley, Hoboken (2011)
Gacanin, H., Ligata, A.: Wi-fi self-organizing networks: challenges and use cases. IEEE Commun. Mag. 55 (7), 158–164 (2017)
Thang, V., Pashchenko, F.: Multistage system-based machine learning techniques for intrusion detection in WiFi network. J. Comput. Netw. Commun. (2019). https://doi.org/10.1155/2019/4708201
Article Google Scholar
Russell, S., Norvig, P.: Artificial Intelligence (a Modern Approach), 3rd edn. Prentice Hall, Hoboken (1995)
MATH Google Scholar
Liu, Y., Bi, S., Shi, Z., Hanzo, L.: When machine learning meets big: a wireless communication perspective. IEEE Veh. Technol. Mag. 15 , 63–72 (2020)
Hu, F., Hao, Q., Bao, K.: A survey on software-defined network and openflow: from concept to implementation. IEEE Commun. Surv. Tutor. 16 , 2181–2206 (2014)
Agarwal, S., Kodialam, M., Lakshman, T.: Traffic engineering in software defined networks. In: Proc. IEEE INFOCOM, pp. 2211–2219 (2013)
Lin, P., Bi, J., Wolff, S.: A west-east bridge based SDN inter-domain testbed. IEEE Commun. Mag. 53 (2), 190–197 (2015)
Xie, J., Yu, F., Huang, T., Xie, R., Liu, J., Wangz, C., Liu, Y.: A survey of machine learning techniques applied to software defined networking (SDN): research issues and challenges. IEEE Commun. Surv. Tutor. 21 , 393–430 (2019)
Kosmidesa, P., Adamopouloua, E., Demestichasa, K., Anagnostoua, M., Rouskasb, A.: On Intelligent Base Station Activation for Next Generation Wireless Networks, the 6th International Conference on Emerging Ubiquitous Systems and Pervasive Networks. Elsevier, Amsterdam (2015)
Li, R., Zhao, Z., Chen, X., Zhang, H.: Energy saving through a learning framework in greener cellular radio access networks. In: Proceedings of GLOBECOM, pp. 1556–1561 (2012)
Ding, H., Zhao, F., Tian, J., Li, D., Zhang, H.: A deep reinforcement learning for user association and power control in heterogeneous net works. Ad Hoc Netw. 102 , 102069 (2020)
Yu, Y., Wang, T., Liew, S.: Deep-reinforcement learning multiple access for heterogeneous wireless networks. IEEE Int. Conf. Commun. (ICC) 37 , 1277–1290 (2018)
Onireti, O.: A cell outage management framework for dense heterogeneous networks. IEEE Trans. Veh. Technol. 65 , 2097–2113 (2016)
Mohammadi, M., Al-Fuqaha, A.: Enabling cognitive smart cities using big data and machine learning: approaches and challenges. IEEE Commun. Mag. 56 , 94–101 (2018)
He, Y.: Software-defined networks with mobile edge computing and caching for smart cities: a big data deep reinforcement learning approach. IEEE Commun. Mag. 55 , 31–37 (2017)
Jia, G., Yang, Z., Lam, H., Shi, J., Shikh-Bahaei, M.: Channel assignment in uplink wireless communication using machine learning approach. IEEE Commun. Lett. 24 , 787–791 (2020)
Zappone, A., Sanguinetti, L., Debbah, M.: User association and load balancing for massive MIMO through deep learning. In: Proceedings of IEEE Asilomar Conference on Signals, Systems, and Computers, pp. 1262–1266 (2018)
Lin, P.: Large-scale and high-dimensional cell outage detection in 5G self-organizing networks. In: Proceedings of APSIPA Annual Summit and Conference, pp. 8–12 (2019)
Pervez, F., Jaber, M., Qadir, J., Younis, S., Imran, M.: Fuzzy Q-learning-based user-centric backhaul-aware user cell association scheme. In: Proceedings of IWCMC, pp. 1840–1845 (2017)
Kumar, Y., Farooq, H., Imran, A.: Fault prediction and reliability analysis in a real cellular network. In: 13th International Wireless Communications and Mobile Computing Conference (IWCMC), pp. 1090–1095 (2017)
Song, Ronggong, Willink, Tricia: Machine Learning-Based Traffic Classification of Wireless Traffic, International Conference on Military Communications and Information Systems (ICMCIS), (2019)
Han, J., Kamber, M., Pei, J.: Data Mining: Concepts and Techniques, 3rd edn. Morgan Kaufmann Publisher, Burlington (2011)
Nguyen, T., Armitage, G., Branch, P., Zander, S.: Timely and continuous machine-learning-based classification for interactive IP traffic. IEEE/ACM Trans. Netw. 20 , 1880–1894 (2012)
Al-Issax, A., Bentaleb, A., Barakabitzex, A., Zinnery, T., Ghita, B.: Bandwidth Prediction Schemes for Defining Bitrate Levels in SDN-enabled Adaptive Streaming, 15th International Conference on Network and Service Management (CNSM) (2019)
Testi, E., Favarelli, E., Giorgetti, A.: Machine Learning For User Traffic Classification in Wireless Systems. 26th European Signal Processing Conference (EUSIPCO) (2018)
Barki, L., Shidling, A., Meti, N., Narayan, D., Mulla, M.: Detection of distributed denial of service attacks in software defined networks. In: Proceedings of IEEE ICACCI, IEEE, pp. 2576–2581 (2016)
Fan, Z., Liu, R.: Investigation of machine learning based network traffic classification. In: Proceedings of ISWCS, pp. 1–6 (2017)
Song, C., Park, Y., Golani, K., Kim, Y., Bhatt, K., Goswami, K.: Machine-learning based threat-aware system in software defined networks. In: Proceedings of IEEE ICCCN, pp. 1–9 (2017)
Glick, M., Rastegarfar, H.: Scheduling and control in hybrid data centers. In: Proceedings IEEE PHOSST’17, pp. 115–116 (2017)
Xiao, P., Qu, W., Qi, H., Xu, Y., Li, Z.: An efficient elephant flow detection with cost-sensitive in SDN. In: Proceedings of IEEE INISCom’15, pp. 24–28, (2015)
Huang, T., Zhang, R., Zhou, C., Sun, L.: QARC: video quality aware rate control for real-time video streaming based on deep reinforcement learning. ACM Multimedia Conference, ACM (2018)
Abdelhadi Azzouni, A., Guy Pujolle, G.: Neutm: A neural network-based framework for traffic matrix prediction in SDN. In: proceedings of the IEEE/IFIP Network Operations and Management Symposium, NOMS (2018). https://doi.org/10.1109/NOMS.2018.8406199
Carner, J., Mestres, A., Alarcn, E., Cabellos, A.: Machine learning-based network modeling: An artificial neural network model vs a theoretical inspired model. In: Proceedings of IEEE ICUFN’17, pp. 522–524 (2017)
Jain, S., Khandelwal, M., Katkar, A., Nygate, J.: Applying big data technologies to manage QoS in an SDN. In: Proceedings of IEEE CNSM’16, pp. 302–306 (2016)
Pasquini, R., Stadler, R.: Learning end-to-end application QoS from OpenFlow switch statistics. In: Proceedings of IEEE NETSOFT’17, pp. 1–9 (2017)
Letaifa, A.: Adaptive QoE monitoring architecture in SDN networks: Video streaming services case. In: Proceedings of IEEE IWCMC’17, pp. 1383–1388 (2017)
Abar, T., Letaifa, A., Asmi, S.: Machine learning based QoE prediction in SDN networks. In: Proceedings of IEEE IWCMC’17, pp. 1395–1400 (2017)
Tayyaba, S., Khattak, H., Almogren, A., Shah, M., Din, I., Alkhalifa, I., Guizani, M.: 5G vehicular network resource management for improving radio access through machine learning. IEEE Access 8 , 6792–6800 (2020)
Comaneci, D., Dobre, C.: Securing networks using SDN and machine learning. In: IEEE International Conference on Computational Science and Engineering (2018)
Murudkar, Chetana V., Gitlin, Richard D.: QoE-driven Anomaly Detection in Self Organizing Mobile Networks Using Machine Learning, 18th annual IEEE Wireless Telecommunications Symposium (WTS) (2019)
Murudkar, C., Gitlin, R.: Machine learning for QoE prediction and anomaly detection in self-organizing mobile networking systems. Int. J. Wirel. Mob. Netw. (IJWMN) (2019). https://doi.org/10.2139/ssrn.3383948
Lim, S.: Software defined network detection system. Int. J. Recent Technol. Eng. (IJRTE) 8 , 1391–1395 (2019)
Yao, H., Mai, T., Xu, X., Zhang, P., Li, M., Liu, Y.: NetworkAI: an intelligent network architecture for self-learning control strategies in software defined networks. IEEE Internet Things J. 5 , 4319–4327 (2018)
Zhu, L., Tang, X., Shen, M., Du, X., Guizani, M.: Privacy-preserving DDoS attack detection using cross-domain traffic in software defined networks. IEEE J. Sel. Areas Commun. 36 , 628–643 (2018)
Côté, D.: Using machine learning in communication networks. J. Opt. Commun. Netw. 10 , D100–D109 (2018)
Gazis, V., Sasloglou, K., Frangiadakis, N., Kikiras, P., Merentitis, A., Mathioudakis, K., Mazarakis, G.: Cooperative communication in channel assignment strategies for IEEE 802.11k WLAN systems. In: IEEE 24th Annual International Symposium on Personal, Indoor, and Mobile Radio Communications (PIMRC), pp. 1924–1929 (2013)
Seyedebrahimi, M., Bouhafs, F., Raschella, A., Mackay, M., Shi, Q.: Fine-grained radio resource management to control interference in dense wi-fi networks. In: IEEE Wireless Communications and Networking Conference (WCNC), pp. 1–6 (2017)
Hartog, F., Raschella, A., Bouhafs, F., Kempker, P., Boltjes, B., Seyedebrahimi, M.: A pathway to solving the wi-fi tragedy of the commons in apartment blocks. In: 27th International Telecommunication Networks and Applications Conference (ITNAC), pp. 1–6 (2017)
Moura, H., Alves, A., Borges, J., Macedo, D., Vieira, M.: Ethanol: a software-defined wireless networking architecture for IEEE 802.11 networks, computer communications, pp. 176–188. Elsevier, Amsterdam (2020)
Lei, T., Wen, X., Lu, Z., Li, Y.: A semi-matching based load balancing scheme for dense IEEE 802.11 WLANs. IEEEIEEE Access 5 , 15332–15339 (2017)
Peng, M., He, G., Wang, L., Kai, C.: AP selection scheme based on achievable throughputs in SDN-enabled WLANs. IEEE Access 7 , 4763–4772 (2019)
Ernst, J., Kremer, S., Rodrigues, J.: A utility based access point selection method for IEEE 802.11 wireless networks with enhanced quality of experience. In: Proceedings of IEEE ICC, pp. 2363–2368 (2014)
Chen, J., Liu, B., Zhou, H., Yu, Q., Gui, L., Shen, X.: QoS-driven efficient client association in high-density software-defined WLAN. IEEE Trans. Veh. Technol. 66 , 7372–7383 (2017)
Bojovic, B., Baldo, N., Nin-Guerrero, J., Dini, P.: A supervised learning approach to cognitive access point selection. In: GLOBECOM Workshops. IEEE, Piscataway (2011)
Wilhelmi, F., Barrachina-Muñnoz, S., Bellalta, B., Cano, C., Jonsson, A., Ram, V.: A flexible machine learning-aware architecture for future WLANs. IEEE Commun. Mag. 58 , 25–31 (2020)
Testolin, A., Zanforlin, M., De Grazia,M., Munaretto, D., Zanella, A., Zorzi, M.: A machine learning approach to qoe-based video admission control and resource allocation in wireless systems. In: Ad Hoc Networking Workshop (MED-HOC-NET), IEEE, pp. 31–38 (2014)
Vassis, D., Kampouraki, A., Belsis, P., Skourlas, C.: Admission control of video sessions over ad hoc networks using neural classifiers. In: IEEE Military Communications Conference, IEEE, pp. 15–20 (2014)
Quer, G., Baldo, N., Zorzi, M.: Cognitive call admission control for voip over ieee 802.11 using bayesian networks. In: Proceedings of GLOBECOM, IEEE, pp. 1–6 (2011)
Coronado, E., Villalon, J., Garrido, A.: Wi-balance: SDN-based load-balancing in enterprise WLANs. In: IEEE Conference on Network Softwarization (NetSoft), pp. 1–2 (2017)
Jagannath, J., Polosky, N., Jagannath, A., Restuccia, F., Melodia, T.: Machine learning for wireless communications in the internet of things: a comprehensive survey. Ad Hoc Netw. 93 , 101913 (2019)
Schmidt, M., Block, D., Meier, U.: Wireless interference identification with convolutional neural networks. In: 15th International Conference on Industrial Informatics (INDIN), IEEE (2017)
Sanguanpuak, T., Guruacharya, S., Rajatheva, N., Bennis, M., Latva-Aho, M.: Multi-operator spectrum sharing for small cell networks: a matching game perspective. IEEE Trans. Wirel. Commun. 16 , 3761–3774 (2017)
Grimaldi, S., Mahmood, A., Gidlund, M.: An SVM-based method for classification of external interference in industrial wireless sensor and actuator networks. J. Sens. Actuator Netw. 6 , 9 (2017)
Kulin, M., Kazaz, T., Moerman, I., Poorter, E.: End-to-end learning from spectrum data: a deep learning approach for wireless signal identification in spectrum monitoring applications. IEEE Access 6 , 18484–18501 (2018)
Davaslioglu, K., Soltani, S., Erpek, T., Sagduyu, Y.: DeepWiFi: cognitive WiFi with deep learning. IEEE Trans. Mob. Comput. 20 , 429–444 (2019)
Jeunen, O., Bosch, P., Herwegen, M., Doorselaer, K., Godman, N., Latre, S.: A machine learning approach for ieee 802.11 channel allocation. In: 14th International Conference on Network and Service Management (CNSM), pp. 28–36 (2018)
Lim, T., Jeon, W., Jeong, D.: Centralized channel allocation scheme in densely deployed 802.11 wireless lans. In: 18th International Conference on Advanced Communication Technology (ICACT), pp. 249–253 (2016)
Baid, A., Raychaudhuri, D.D.: Understanding channel selection dynamics in dense Wi-Fi networks. IEEE Commun. Mag. 53 , 110–117 (2015)
Moura, H., Macedo, D., Vieira, M.: Automatic quality of experience management for wlan networks using multi-armed bandit. In: IFIP/IEEE Symposium on Integrated Network and Service Management (IM), pp. 279–288 (2019)
Singh, S.: SDN (Software Defined Network) and Machine Learning for High-Density WLANs. In: Proceedings of National Conference on Machine Learning, pp. 82–91 (2019)
Herzen, J., Lundgren, H., Hegde, N.: Learning Wi-Fi Performance, 12th Annual International Conference on Sensing, Communication, and Networking (SECON), IEEE (2015)
Boutaba, R., Salahuddin, M., Limam, N., Ayoubi, S., Shahriar, N., Estrada-Solano, F., Caicedo, O.: A comprehensive survey on machine learning for networking: evolution, applications and research opportunities. J. Internet Serv. Appl. 9 , 1–99 (2018)
Han, K., Lee, J., Kim, B.: Machine-Learning based Loss Discrimination Algorithm for Wireless TCP Congestion Control. International Conference on Electronics, Information, and Communication (ICEIC) (2019)
Moriyama, Tomokazu, Yamamoto, Ryo, Ohzahata, Satoshi, Kato, Toshihiko: TCP Congestion Control over IEEE 802.11 Wireless Lans based on K-Means Clustering Focusing on Congestion Window Size and Round-trip Time. International Conference on Data Communication Networking (2018)
Sui, K., Zhou, M., Liu, D., Ma, M., Pei, D., Zhao, Y., Li, Z., Moscibroda, T.: Characterizing and Improving WiFi Latency in Large-Scale Operational Networks, The 14th ACM International Conference on Mobile Systems, Applications, and Services, ACM (2016)
Coronado, E., Thomas, A., Riggio, R.: Adaptive ML-based frame length optimization in enterprise SD-WLANs. J. Netw. Syst. Manage. (2020). https://doi.org/10.1007/s10922-020-09527-y
Ibarrola, E., Davis, M., Voisin, C., Close, C., Cristobo, L.: QoE enhancement in next generation wireless ecosystems: a machine learning approach. IEEE Commun. Stand. Mag. 3 , 63–70 (2019)
Košťál, K., Bencel, R., Ries, M., Trúchly, P., Kotuliak, I.: High performance SDN WLAN architecture, Sensors. In: Proceedings of PMC (2019)
Wang, Z., Xu, Y., Li, L., Tian, H., Cui, S.: Handover control in wireless systems via asynchronous multi-user deep reinforcement learning. IEEE Internet Things J. 5 , 4296–4307 (2018)
Zhou, P., Chang, Y., Copeland, J.: Determination of wireless networks parameters through parallel hierarchical support vector machines. IEEE Trans. Parallel Distrib. Syst. 23 , 505–512 (2012)
Yu, C., Chen, K., Cheng, S.: Cognitive radio network tomography. IEEE Trans. Veh. Technol. 59 , 1980–1997 (2010)
Xia, M.: Optical and wireless hybrid access networks: design and optimization. OSA/IEEE J. Opt. Commun. Netw. 4 , 749–759 (2012)
Sequeira, L., Cruz, J., Ruiz-Mas, J., Saldana, J., Fernandez-Navajas, J., Almodovar, J.: Building an SDN enterprise WLAN based on virtual APs. IEEE Commun. Lett. 21 , 374–377 (2017)
Download references
Author information
Authors and affiliations.
Palestine Polytechnic University, Hebron, Palestine
Murad Abusubaih
You can also search for this author in PubMed Google Scholar
Corresponding author
Correspondence to Murad Abusubaih .
Additional information
Publisher's note.
Springer Nature remains neutral with regard to jurisdictional claims in published maps and institutional affiliations.

Rights and permissions
Reprints and permissions
About this article
Abusubaih, M. Intelligent Wireless Networks: Challenges and Future Research Topics. J Netw Syst Manage 30 , 18 (2022). https://doi.org/10.1007/s10922-021-09625-5
Download citation
Received : 07 July 2020
Revised : 18 May 2021
Accepted : 22 August 2021
Published : 21 October 2021
DOI : https://doi.org/10.1007/s10922-021-09625-5
Share this article
Anyone you share the following link with will be able to read this content:
Sorry, a shareable link is not currently available for this article.
Provided by the Springer Nature SharedIt content-sharing initiative
- Artificial intelligence
- Machine learning
- Network management
- Network optimization
- Find a journal
- Publish with us
- Track your research
Academia.edu no longer supports Internet Explorer.
To browse Academia.edu and the wider internet faster and more securely, please take a few seconds to upgrade your browser .
- We're Hiring!
- Help Center
Wireless Network
- Most Cited Papers
- Most Downloaded Papers
- Newest Papers
- Last »
- Missing Data Follow Following
- Cellular Network Follow Following
- Coronary Artery Disease Follow Following
- Mathematical Analysis Follow Following
- Performance Evaluation Follow Following
- Standardisation Follow Following
- Middleware Follow Following
- Smart Antenna Follow Following
- Gender Difference Follow Following
- Personal Follow Following
Enter the email address you signed up with and we'll email you a reset link.
- Academia.edu Journals
- We're Hiring!
- Help Center
- Find new research papers in:
- Health Sciences
- Earth Sciences
- Cognitive Science
- Mathematics
- Computer Science
- Academia ©2024
- wireless network research topics
Wireless network is referred to as a computer network, which is an intriguing as well as rapidly evolving domain. Relevant to wireless networks, we suggest numerous research topics that could be appropriate for your thesis or project work:
- 5G and Beyond:
- Topic: Performance Optimization in 5G Networks
- Explanation: Consider various metrics like energy effectiveness, throughput, and latency to improve the performance of 5G networks through exploring methods.
- Major Areas: Edge computing, network slicing, beamforming, and massive MIMO.
- Topic: Integration of 5G and IoT for Smart Cities
- Explanation: To create smart city applications, in what way 5G mechanism can be combined with IoT has to be analyzed.
- Major Areas: Ecological tracking, traffic handling, and smart architecture.
- 6G Networks:
- Topic: Exploring Terahertz Communication for 6G Networks
- Explanation: In utilizing terahertz frequencies for ultra-high-speed wireless interaction, examine the problems and effectiveness.
- Major Areas: Modulation approaches, hardware development, and propagation features.
- Topic: Intelligent Reflecting Surfaces (IRS) for 6G
- Explanation: To improve network coverage and signal propagation in 6G, the application of IRS must be explored.
- Major Areas: IRS model, enhancement, and application.
- Cognitive Radio Networks:
- Topic: Dynamic Spectrum Access in Cognitive Radio Networks
- Explanation: For dynamic spectrum access and effective spectrum sensing, create and assess methods.
- Major Areas: Interference handling, spectrum prediction with machine learning, and spectrum sensing approaches.
- Vehicular Ad-Hoc Networks (VANETs):
- Topic: Secure Communication Protocols for Autonomous Vehicles
- Explanation: Among self-driving vehicles, assure credible and safer interaction by modeling protocols.
- Major Areas: Authentication, encryption, and V2I (vehicle-to-infrastructure) and V2V (vehicle-to-vehicle) interaction.
- Topic: Enhancing Safety Applications in VANETs
- Explanation: Aim to create efficient applications, which enhance traffic handling and road safety through the use of VANETs.
- Major Areas: Actual-time traffic monitoring and collision avoidance systems.
- Internet of Things (IoT):
- Topic: Energy-Efficient Protocols for IoT Networks
- Explanation: In order to extend the battery durability of IoT devices, create protocols that are capable of reducing power usage in these devices.
- Major Areas: Sleep scheduling, data aggregation approaches, and MAC layer protocols.
- Topic: Security Solutions for IoT Networks
- Explanation: Appropriate for resource-limited IoT devices, the lightweight security techniques have to be created.
- Major Areas: Intrusion detection systems, authentication, and encryption.
- Mobile Edge Computing (MEC):
- Topic: Enhancing Mobile Application Performance with Edge Computing
- Explanation: To enhance the performance of mobile applications and minimize latency, employ edge computing.
- Major Areas: Actual-time data processing, resource allocation, and task offloading.
- Wireless Sensor Networks (WSNs):
- Topic: Robust Data Aggregation Techniques in WSNs
- Explanation: In spite of node faults, assure credible data aggregation in sensor networks by creating approaches.
- Major Areas: Data morality, energy effectiveness, and fault tolerance.
- Topic: Security Mechanisms for WSNs
- Explanation: As a means to secure data sharing in WSNs against different hazards, apply security protocols.
- Major Areas: Secure routing, key handling, and encryption.
- Software-Defined Networking (SDN):
- Topic: SDN-Based Network Management for Wireless Networks
- Explanation: To enhance and handle the wireless networks performance, utilize SDN.
- Major Areas: Traffic engineering, network virtualization, and dynamic resource allocation.
- Topic: Enhancing Security in SDN-Enabled Wireless Networks
- Explanation: In order to secure SDN-related wireless networks against assaults, create security techniques.
- Major Areas: Access control, secure interaction, and intrusion detection.
- Machine Learning in Wireless Networks:
- Topic: AI-Driven Network Management and Optimization
- Explanation: To handle resources and improve network performance in an effective manner, implement the methods of machine learning.
- Major Areas: Dynamic resource handling, anomaly identification, and traffic forecasting.
- Topic: Machine Learning for Anomaly Detection in Wireless Networks
- Explanation: In wireless networks, identify and react to possible safety hazards and abnormalities by creating machine learning frameworks.
- Major Areas: Actual-time anomaly identification, and supervised and unsupervised learning.
- Visible Light Communication (VLC):
- Topic: High-Speed Data Transmission Using VLC
- Explanation: Specifically for extensive-speed data sharing in indoor platforms, create VLC systems.
- Major Areas: Interference reduction, system design, and modulation approaches.
- Blockchain for Wireless Networks:
- Topic: Blockchain-Based Security Framework for IoT Networks
- Explanation: Improve confidentiality and safety in IoT networks through the utilization of blockchain mechanisms.
- Major Areas: Smart contracts, data morality, and decentralized authentication.
- Energy Harvesting and Green Communication:
- Topic: Sustainable Energy Solutions for Wireless Sensor Networks
- Explanation: To expand the durability of wireless sensor networks, investigate energy harvesting approaches.
- Major Areas: Piezoelectric, RF, and solar energy harvesting techniques.
- Quantum Communication:
- Topic: Quantum Key Distribution for Secure Wireless Communication
- Explanation: In wireless interaction systems, improve security by applying QKD protocols.
- Major Areas: Secure key handling, photon loss management, and error rectification.
What are some examples of simulation tools for wireless network projects?
There are several simulation tools useful for wireless network projects. In terms of project requirements, a suitable simulation tool has to be selected. Appropriate for wireless network projects, we list out a few instances of simulation tools which are utilized extensively:
- NS-3 (Network Simulator 3)
- Outline: NS-3 is majorly employed for academic and research objectives. It is referred to as an open-source discrete-event network simulator.
- Characteristics: It enables the simulation of IoT and 5G networks, offers models for wired and wireless networks, and facilitates enormous network protocols.
- Application Areas: This simulator is more suitable for protocol creation, performance analysis, and simulation of extensive network topologies.
- Outline: OMNeT++ is considered as a flexible, component-related C++ simulation framework and library.
- Characteristics: It encompasses architectures such as INET for internet simulations, enables different network protocols, and is more adaptable.
- Application Areas: Highly ideal for network performance assessment, traffic designing, and simulation of communication networks.
- Outline: For modeling, simulating, and examining interaction networks, QualNet offers an extensive platform. It is generally a commercial network simulation software.
- Characteristics: It supports a broad range of protocol libraries, visualization tools, and actual-time network simulation.
- Application Areas: High-fidelity simulations of wireless networks are supported by QualNet. These networks include sensor networks and mobile ad-hoc networks (MANETs).
- MATLAB and Simulink
- Outline: It is examined as a graphical simulation tool and high-level programming platform.
- Characteristics: For wireless communication, system designing, and signal processing, it offers a wide range of toolboxes.
- Application Areas: More suitable for examining network performance, creating and assessing methods, and simulation of communication systems.
- OPNET (now phase of Riverbed Modeler)
- Outline: OPNET is primarily utilized for designing, enhancing, and engineering networks. It is considered as a network designing and simulation tool.
- Characteristics: It enables performance analysis tools, extensive protocols, and in-depth designing of network devices.
- Application Areas: This tool is highly appropriate for the simulation of complicated communication systems, wireless LANs, and enterprise networks.
- GNS3 (Graphical Network Simulator 3)
- Outline: GNS3 is an efficient network software emulator. To simulate complicated networks, it enables the integration of actual and virtual devices.
- Characteristics: This emulator facilitates combination with actual network hardware and enables extensive network software and hardware.
- Application Areas: It is majorly suitable for protocol creation, network modeling, setup, and assessment.
- Riverbed Modeler (formerly OPNET)
- Outline: For performance analysis, network planning, and structure, Riverbed Modeler is very helpful. It is a robust network simulation and designing tool.
- Characteristics: This tool supports in-depth simulation of network protocols, performance enhancement, and traffic analysis.
- Application Areas: Simulation of complicated wireless networks is enabled by Riverbed modeler. It encompasses IoT networks, MANETs, and cellular networks.
- Outline: NetSim is specifically a network simulation tool, appropriate for the simulation of wireless and wired networks, and designing of protocol.
- Characteristics: It involves an excellent graphical interface, offers in-depth performance metrics, and enables different network protocols.
- Application Areas: This tool is more appropriate for network protocol creation, research projects, and academic objectives.
- Cooja (Contiki OS Simulator)
- Outline: Cooja is a phase of the Contiki OS ecosystem. It is generally a network simulator modeled for sensor and IoT networks.
- Characteristics: It offers elaborate visualization, enables low-power interaction protocols, and simulates different hardware environments.
- Application Areas: This simulator is highly ideal for energy usage analysis, protocol creation, and simulations of wireless sensor network and IoT.
- TinyOS and TOSSIM
- Outline: TinyOS is more useful for low-power wireless devices. It is a freely accessible operating system. TOSSIM is specified as the simulator of TinyOS.
- Characteristics: It enables different interaction protocols, offers in-depth logging, and simulates extensive wireless sensor networks.
- Application Areas: To create and assess protocols for IoT devices and wireless sensor networks, it is highly suitable.
- Outline: Castalia is an effective simulator relevant to the OMNeT++ environment. It is majorly useful for body area networks and wireless sensor networks.
- Characteristics: This simulator facilitates different network and MAC layer protocols, and designs practical wireless channel and radio activity.
- Application Areas: It is more appropriate for application creation, performance assessment, and exploring sensor network protocols.
- Outline: EstiNet enables wireless as well as wired networks. It is specifically a network emulation and simulation tool.
- Characteristics: It supports combination with network hardware, provides in-depth protocol designs, and allows actual-time simulation.
- Application Areas: This tool is suitable for network model, performance analysis, and simulation and emulation of network protocols.
Wireless Network Research Ideas
Generating research ideas for a wireless network independently is a challenging endeavor. However, our team of experts possesses extensive practical experience of over 17+ years in this field. When approached by a scholar, we request them to provide us with all the essential information regarding their ideas, including base paper or reference paper details. Subsequently, we commence our work by thoroughly examining all aspects of the proposed research.
- Empowering full-duplex wireless communication by exploiting directional diversity
- On the distribution of the sum of gamma-gamma variates and applications in RF and optical wireless communications
- Hybrid PLC/wireless communication for smart grids and internet of things applications
- Qualitative theory of dynamical systems, chaos and contemporary wireless communications
- Performance bounds of multihop wireless communications with blind relays over generalized fading channels
- Channel equalization in filter bank based multicarrier modulation for wireless communications
- Sensitive and nonlinear far-field RF energy harvesting in wireless communications
- Research and development of microwave dielectric ceramics for wireless communications
- An easy to deploy street light control system based on wireless communication and LED technology
- A network information theory for wireless communication: Scaling laws and optimal operation
- Statistics of the sum of lognormal variables in wireless communications
- A new compact filter-antenna for modern wireless communication systems
- Overview of channel models for underwater wireless communication networks
- Synthesis of an electromagnetic wave absorber for high-speed wireless communication
- On the design of a solar-panel receiver for optical wireless communications with simultaneous energy harvesting
- Graphene-enabled wireless communication for massive multicore architectures
- Space-time codes for high data rate wireless communication: performance criteria in the presence of channel estimation errors, mobility, and multiple paths
- A robust voice activity detector for wireless communications using soft computing
- Deep learning-based end-to-end wireless communication systems with conditional GANs as unknown channels
- Integrated energy and spectrum harvesting for 5G wireless communications
Research on Wireless Network and Its Technology
Ieee account.
- Change Username/Password
- Update Address
Purchase Details
- Payment Options
- Order History
- View Purchased Documents
Profile Information
- Communications Preferences
- Profession and Education
- Technical Interests
- US & Canada: +1 800 678 4333
- Worldwide: +1 732 981 0060
- Contact & Support
- About IEEE Xplore
- Accessibility
- Terms of Use
- Nondiscrimination Policy
- Privacy & Opting Out of Cookies
A not-for-profit organization, IEEE is the world's largest technical professional organization dedicated to advancing technology for the benefit of humanity. © Copyright 2024 IEEE - All rights reserved. Use of this web site signifies your agreement to the terms and conditions.

COMMENTS
A wireless network refers to any type of computer network that is not connected by cables of any kind. | Explore the latest full-text research PDFs, articles, conference papers, preprints and more ...
Recently, artificial intelligence (AI) has become a primary tool of serving science and humanity in all fields. This is due to the significant development in computing. The use of AI and machine learning (ML) has extended to wireless networks that are constantly evolving. This enables better operation and management of networks, through algorithms that learn and utilize available data and ...
This paper focuses on assessing existing and new threats, possible areas of vulnerability in the wireless network system, and probable solutions to curb the threats. View Show abstract
wireless communication of network optimization, handover, and interference should be able to exploit the concepts of big data to facilitate these operations. Providing other add-ons such as
Tahani Alnemran Journal of Engineering Research and Application ISSN: 2248-9622 Vol. 10, Issue 01 (Series -III) January 2020, pp 23-27 RESEARCH ARTICLE www.ijera.com OPEN ACCESS Wireless Networks: Types, Implementations, and Applications Tahani Alnemran and Mahnaz Qabazard The Higher Institue Of Telecommunication&Navigation Corresponding Author: Tahani Alnemran ----- -----Date of Submission ...
Recent papers in Wireless Network. Top Papers; Most Cited Papers; Most Downloaded Papers; ... This topic deals with cutting-edge research in various aspects related to the theory and practice of mobile computing or wireless and mobile networking. These aspects include architectures, algorithms, networks, protocols, modeling and performance ...
The work in emphasized enhancements in the multilevel architecture through the integration of AI in URLLC, offering a novel approach to wireless network design. Additionally, this research paper discussed existing multilevel architectures and provided further ideas on several research gaps using DL in 6G networks.
The demand for wireless connectivity has grown exponentially over the last few decades. Fifth-generation (5G) communications, with far more features than fourth-generation communications, will soon be deployed worldwide. A new paradigm of wireless communication, the sixth-generation (6G) system, with the full support of artificial intelligence, is expected to be implemented between 2027 and ...
Generating research ideas for a wireless network independently is a challenging endeavor. However, our team of experts possesses extensive practical experience of over 17+ years in this field. When approached by a scholar, we request them to provide us with all the essential information regarding their ideas, including base paper or reference ...
Wireless and mobile networks represent an active research and new technology development area. In this paper we analyze wireless network and discuss the applications of wireless technology. To fully support mobility, a next generation Internet must provide ways to name and route to a much richer set of network elements than just attachment points. It should support routing in terms of names ...