Education reform and change driven by digital technology: a bibliometric study from a global perspective
- Chengliang Wang 1 ,
- Xiaojiao Chen 1 ,
- Teng Yu ORCID: orcid.org/0000-0001-5198-7261 2 , 3 ,
- Yidan Liu 1 , 4 &
- Yuhui Jing 1
Humanities and Social Sciences Communications volume 11 , Article number: 256 ( 2024 ) Cite this article
23k Accesses
18 Citations
10 Altmetric
Metrics details
- Development studies
- Science, technology and society
Amidst the global digital transformation of educational institutions, digital technology has emerged as a significant area of interest among scholars. Such technologies have played an instrumental role in enhancing learner performance and improving the effectiveness of teaching and learning. These digital technologies also ensure the sustainability and stability of education during the epidemic. Despite this, a dearth of systematic reviews exists regarding the current state of digital technology application in education. To address this gap, this study utilized the Web of Science Core Collection as a data source (specifically selecting the high-quality SSCI and SCIE) and implemented a topic search by setting keywords, yielding 1849 initial publications. Furthermore, following the PRISMA guidelines, we refined the selection to 588 high-quality articles. Using software tools such as CiteSpace, VOSviewer, and Charticulator, we reviewed these 588 publications to identify core authors (such as Selwyn, Henderson, Edwards), highly productive countries/regions (England, Australia, USA), key institutions (Monash University, Australian Catholic University), and crucial journals in the field ( Education and Information Technologies , Computers & Education , British Journal of Educational Technology ). Evolutionary analysis reveals four developmental periods in the research field of digital technology education application: the embryonic period, the preliminary development period, the key exploration, and the acceleration period of change. The study highlights the dual influence of technological factors and historical context on the research topic. Technology is a key factor in enabling education to transform and upgrade, and the context of the times is an important driving force in promoting the adoption of new technologies in the education system and the transformation and upgrading of education. Additionally, the study identifies three frontier hotspots in the field: physical education, digital transformation, and professional development under the promotion of digital technology. This study presents a clear framework for digital technology application in education, which can serve as a valuable reference for researchers and educational practitioners concerned with digital technology education application in theory and practice.

Similar content being viewed by others
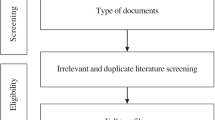
A bibliometric analysis of knowledge mapping in Chinese education digitalization research from 2012 to 2022
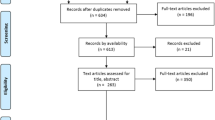
Digital transformation and digital literacy in the context of complexity within higher education institutions: a systematic literature review
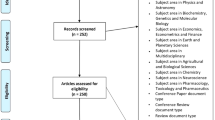
Education big data and learning analytics: a bibliometric analysis
Introduction.
Digital technology has become an essential component of modern education, facilitating the extension of temporal and spatial boundaries and enriching the pedagogical contexts (Selwyn and Facer, 2014 ). The advent of mobile communication technology has enabled learning through social media platforms (Szeto et al. 2015 ; Pires et al. 2022 ), while the advancement of augmented reality technology has disrupted traditional conceptions of learning environments and spaces (Perez-Sanagustin et al., 2014 ; Kyza and Georgiou, 2018 ). A wide range of digital technologies has enabled learning to become a norm in various settings, including the workplace (Sjöberg and Holmgren, 2021 ), home (Nazare et al. 2022 ), and online communities (Tang and Lam, 2014 ). Education is no longer limited to fixed locations and schedules, but has permeated all aspects of life, allowing learning to continue at any time and any place (Camilleri and Camilleri, 2016 ; Selwyn and Facer, 2014 ).
The advent of digital technology has led to the creation of several informal learning environments (Greenhow and Lewin, 2015 ) that exhibit divergent form, function, features, and patterns in comparison to conventional learning environments (Nygren et al. 2019 ). Consequently, the associated teaching and learning processes, as well as the strategies for the creation, dissemination, and acquisition of learning resources, have undergone a complete overhaul. The ensuing transformations have posed a myriad of novel issues, such as the optimal structuring of teaching methods by instructors and the adoption of appropriate learning strategies by students in the new digital technology environment. Consequently, an examination of the principles that underpin effective teaching and learning in this environment is a topic of significant interest to numerous scholars engaged in digital technology education research.
Over the course of the last two decades, digital technology has made significant strides in the field of education, notably in extending education time and space and creating novel educational contexts with sustainability. Despite research attempts to consolidate the application of digital technology in education, previous studies have only focused on specific aspects of digital technology, such as Pinto and Leite’s ( 2020 ) investigation into digital technology in higher education and Mustapha et al.’s ( 2021 ) examination of the role and value of digital technology in education during the pandemic. While these studies have provided valuable insights into the practical applications of digital technology in particular educational domains, they have not comprehensively explored the macro-mechanisms and internal logic of digital technology implementation in education. Additionally, these studies were conducted over a relatively brief period, making it challenging to gain a comprehensive understanding of the macro-dynamics and evolutionary process of digital technology in education. Some studies have provided an overview of digital education from an educational perspective but lack a precise understanding of technological advancement and change (Yang et al. 2022 ). Therefore, this study seeks to employ a systematic scientific approach to collate relevant research from 2000 to 2022, comprehend the internal logic and development trends of digital technology in education, and grasp the outstanding contribution of digital technology in promoting the sustainability of education in time and space. In summary, this study aims to address the following questions:
RQ1: Since the turn of the century, what is the productivity distribution of the field of digital technology education application research in terms of authorship, country/region, institutional and journal level?
RQ2: What is the development trend of research on the application of digital technology in education in the past two decades?
RQ3: What are the current frontiers of research on the application of digital technology in education?
Literature review
Although the term “digital technology” has become ubiquitous, a unified definition has yet to be agreed upon by scholars. Because the meaning of the word digital technology is closely related to the specific context. Within the educational research domain, Selwyn’s ( 2016 ) definition is widely favored by scholars (Pinto and Leite, 2020 ). Selwyn ( 2016 ) provides a comprehensive view of various concrete digital technologies and their applications in education through ten specific cases, such as immediate feedback in classes, orchestrating teaching, and community learning. Through these specific application scenarios, Selwyn ( 2016 ) argues that digital technology encompasses technologies associated with digital devices, including but not limited to tablets, smartphones, computers, and social media platforms (such as Facebook and YouTube). Furthermore, Further, the behavior of accessing the internet at any location through portable devices can be taken as an extension of the behavior of applying digital technology.
The evolving nature of digital technology has significant implications in the field of education. In the 1890s, the focus of digital technology in education was on comprehending the nuances of digital space, digital culture, and educational methodologies, with its connotations aligned more towards the idea of e-learning. The advent and subsequent widespread usage of mobile devices since the dawn of the new millennium have been instrumental in the rapid expansion of the concept of digital technology. Notably, mobile learning devices such as smartphones and tablets, along with social media platforms, have become integral components of digital technology (Conole and Alevizou, 2010 ; Batista et al. 2016 ). In recent times, the burgeoning application of AI technology in the education sector has played a vital role in enriching the digital technology lexicon (Banerjee et al. 2021 ). ChatGPT, for instance, is identified as a novel educational technology that has immense potential to revolutionize future education (Rospigliosi, 2023 ; Arif, Munaf and Ul-Haque, 2023 ).
Pinto and Leite ( 2020 ) conducted a comprehensive macroscopic survey of the use of digital technologies in the education sector and identified three distinct categories, namely technologies for assessment and feedback, mobile technologies, and Information Communication Technologies (ICT). This classification criterion is both macroscopic and highly condensed. In light of the established concept definitions of digital technology in the educational research literature, this study has adopted the characterizations of digital technology proposed by Selwyn ( 2016 ) and Pinto and Leite ( 2020 ) as crucial criteria for analysis and research inclusion. Specifically, this criterion encompasses several distinct types of digital technologies, including Information and Communication Technologies (ICT), Mobile tools, eXtended Reality (XR) Technologies, Assessment and Feedback systems, Learning Management Systems (LMS), Publish and Share tools, Collaborative systems, Social media, Interpersonal Communication tools, and Content Aggregation tools.
Methodology and materials
Research method: bibliometric.
The research on econometric properties has been present in various aspects of human production and life, yet systematic scientific theoretical guidance has been lacking, resulting in disorganization. In 1969, British scholar Pritchard ( 1969 ) proposed “bibliometrics,” which subsequently emerged as an independent discipline in scientific quantification research. Initially, Pritchard defined bibliometrics as “the application of mathematical and statistical methods to books and other media of communication,” however, the definition was not entirely rigorous. To remedy this, Hawkins ( 2001 ) expanded Pritchard’s definition to “the quantitative analysis of the bibliographic features of a body of literature.” De Bellis further clarified the objectives of bibliometrics, stating that it aims to analyze and identify patterns in literature, such as the most productive authors, institutions, countries, and journals in scientific disciplines, trends in literary production over time, and collaboration networks (De Bellis, 2009 ). According to Garfield ( 2006 ), bibliometric research enables the examination of the history and structure of a field, the flow of information within the field, the impact of journals, and the citation status of publications over a longer time scale. All of these definitions illustrate the unique role of bibliometrics as a research method for evaluating specific research fields.
This study uses CiteSpace, VOSviewer, and Charticulator to analyze data and create visualizations. Each of these three tools has its own strengths and can complement each other. CiteSpace and VOSviewer use set theory and probability theory to provide various visualization views in fields such as keywords, co-occurrence, and co-authors. They are easy to use and produce visually appealing graphics (Chen, 2006 ; van Eck and Waltman, 2009 ) and are currently the two most widely used bibliometric tools in the field of visualization (Pan et al. 2018 ). In this study, VOSviewer provided the data necessary for the Performance Analysis; Charticulator was then used to redraw using the tabular data exported from VOSviewer (for creating the chord diagram of country collaboration); this was to complement the mapping process, while CiteSpace was primarily utilized to generate keyword maps and conduct burst word analysis.
Data retrieval
This study selected documents from the Science Citation Index Expanded (SCIE) and Social Science Citation Index (SSCI) in the Web of Science Core Collection as the data source, for the following reasons:
(1) The Web of Science Core Collection, as a high-quality digital literature resource database, has been widely accepted by many researchers and is currently considered the most suitable database for bibliometric analysis (Jing et al. 2023a ). Compared to other databases, Web of Science provides more comprehensive data information (Chen et al. 2022a ), and also provides data formats suitable for analysis using VOSviewer and CiteSpace (Gaviria-Marin et al. 2019 ).
(2) The application of digital technology in the field of education is an interdisciplinary research topic, involving technical knowledge literature belonging to the natural sciences and education-related literature belonging to the social sciences. Therefore, it is necessary to select Science Citation Index Expanded (SCIE) and Social Science Citation Index (SSCI) as the sources of research data, ensuring the comprehensiveness of data while ensuring the reliability and persuasiveness of bibliometric research (Hwang and Tsai, 2011 ; Wang et al. 2022 ).
After establishing the source of research data, it is necessary to determine a retrieval strategy (Jing et al. 2023b ). The choice of a retrieval strategy should consider a balance between the breadth and precision of the search formula. That is to say, it should encompass all the literature pertaining to the research topic while excluding irrelevant documents as much as possible. In light of this, this study has set a retrieval strategy informed by multiple related papers (Mustapha et al. 2021 ; Luo et al. 2021 ). The research by Mustapha et al. ( 2021 ) guided us in selecting keywords (“digital” AND “technolog*”) to target digital technology, while Luo et al. ( 2021 ) informed the selection of terms (such as “instruct*,” “teach*,” and “education”) to establish links with the field of education. Then, based on the current application of digital technology in the educational domain and the scope of selection criteria, we constructed the final retrieval strategy. Following the general patterns of past research (Jing et al. 2023a , 2023b ), we conducted a specific screening using the topic search (Topics, TS) function in Web of Science. For the specific criteria used in the screening for this study, please refer to Table 1 .
Literature screening
Literature acquired through keyword searches may contain ostensibly related yet actually unrelated works. Therefore, to ensure the close relevance of literature included in the analysis to the research topic, it is often necessary to perform a manual screening process to identify the final literature to be analyzed, subsequent to completing the initial literature search.
The manual screening process consists of two steps. Initially, irrelevant literature is weeded out based on the title and abstract, with two members of the research team involved in this phase. This stage lasted about one week, resulting in 1106 articles being retained. Subsequently, a comprehensive review of the full text is conducted to accurately identify the literature required for the study. To carry out the second phase of manual screening effectively and scientifically, and to minimize the potential for researcher bias, the research team established the inclusion criteria presented in Table 2 . Three members were engaged in this phase, which took approximately 2 weeks, culminating in the retention of 588 articles after meticulous screening. The entire screening process is depicted in Fig. 1 , adhering to the PRISMA guidelines (Page et al. 2021 ).
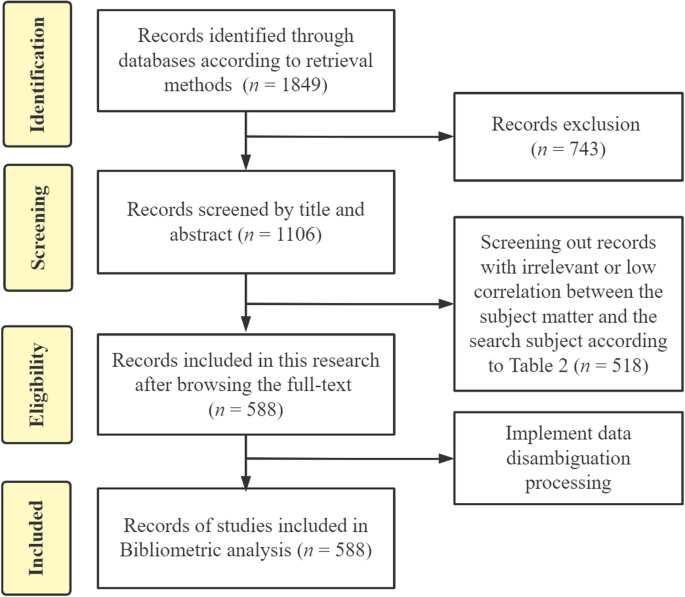
The process of obtaining and filtering the necessary literature data for research.
Data standardization
Nguyen and Hallinger ( 2020 ) pointed out that raw data extracted from scientific databases often contains multiple expressions of the same term, and not addressing these synonymous expressions could affect research results in bibliometric analysis. For instance, in the original data, the author list may include “Tsai, C. C.” and “Tsai, C.-C.”, while the keyword list may include “professional-development” and “professional development,” which often require merging. Therefore, before analyzing the selected literature, a data disambiguation process is necessary to standardize the data (Strotmann and Zhao, 2012 ; Van Eck and Waltman, 2019 ). This study adopted the data standardization process proposed by Taskin and Al ( 2019 ), mainly including the following standardization operations:
Firstly, the author and source fields in the data are corrected and standardized to differentiate authors with similar names.
Secondly, the study checks whether the journals to which the literature belongs have been renamed in the past over 20 years, so as to avoid the influence of periodical name change on the analysis results.
Finally, the keyword field is standardized by unifying parts of speech and singular/plural forms of keywords, which can help eliminate redundant entries in the knowledge graph.
Performance analysis (RQ1)
This section offers a thorough and detailed analysis of the state of research in the field of digital technology education. By utilizing descriptive statistics and visual maps, it provides a comprehensive overview of the development trends, authors, countries, institutions, and journal distribution within the field. The insights presented in this section are of great significance in advancing our understanding of the current state of research in this field and identifying areas for further investigation. The use of visual aids to display inter-country cooperation and the evolution of the field adds to the clarity and coherence of the analysis.
Time trend of the publications
To understand a research field, it is first necessary to understand the most basic quantitative information, among which the change in the number of publications per year best reflects the development trend of a research field. Figure 2 shows the distribution of publication dates.
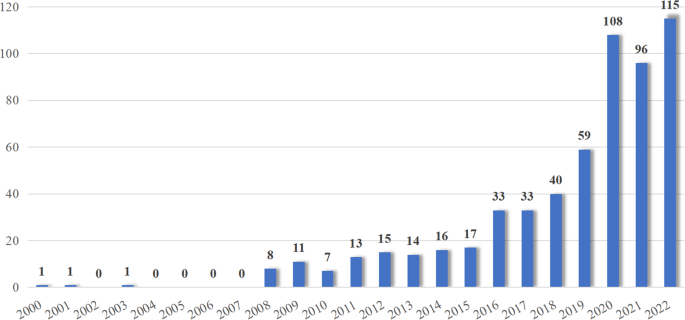
Time trend of the publications on application of digital technology in education.
From the Fig. 2 , it can be seen that the development of this field over the past over 20 years can be roughly divided into three stages. The first stage was from 2000 to 2007, during which the number of publications was relatively low. Due to various factors such as technological maturity, the academic community did not pay widespread attention to the role of digital technology in expanding the scope of teaching and learning. The second stage was from 2008 to 2019, during which the overall number of publications showed an upward trend, and the development of the field entered an accelerated period, attracting more and more scholars’ attention. The third stage was from 2020 to 2022, during which the number of publications stabilized at around 100. During this period, the impact of the pandemic led to a large number of scholars focusing on the role of digital technology in education during the pandemic, and research on the application of digital technology in education became a core topic in social science research.
Analysis of authors
An analysis of the author’s publication volume provides information about the representative scholars and core research strengths of a research area. Table 3 presents information on the core authors in adaptive learning research, including name, publication number, and average number of citations per article (based on the analysis and statistics from VOSviewer).
Variations in research foci among scholars abound. Within the field of digital technology education application research over the past two decades, Neil Selwyn stands as the most productive author, having published 15 papers garnering a total of 1027 citations, resulting in an average of 68.47 citations per paper. As a Professor at the Faculty of Education at Monash University, Selwyn concentrates on exploring the application of digital technology in higher education contexts (Selwyn et al. 2021 ), as well as related products in higher education such as Coursera, edX, and Udacity MOOC platforms (Bulfin et al. 2014 ). Selwyn’s contributions to the educational sociology perspective include extensive research on the impact of digital technology on education, highlighting the spatiotemporal extension of educational processes and practices through technological means as the greatest value of educational technology (Selwyn, 2012 ; Selwyn and Facer, 2014 ). In addition, he provides a blueprint for the development of future schools in 2030 based on the present impact of digital technology on education (Selwyn et al. 2019 ). The second most productive author in this field, Henderson, also offers significant contributions to the understanding of the important value of digital technology in education, specifically in the higher education setting, with a focus on the impact of the pandemic (Henderson et al. 2015 ; Cohen et al. 2022 ). In contrast, Edwards’ research interests focus on early childhood education, particularly the application of digital technology in this context (Edwards, 2013 ; Bird and Edwards, 2015 ). Additionally, on the technical level, Edwards also mainly prefers digital game technology, because it is a digital technology that children are relatively easy to accept (Edwards, 2015 ).
Analysis of countries/regions and organization
The present study aimed to ascertain the leading countries in digital technology education application research by analyzing 75 countries related to 558 works of literature. Table 4 depicts the top ten countries that have contributed significantly to this field in terms of publication count (based on the analysis and statistics from VOSviewer). Our analysis of Table 4 data shows that England emerged as the most influential country/region, with 92 published papers and 2401 citations. Australia and the United States secured the second and third ranks, respectively, with 90 papers (2187 citations) and 70 papers (1331 citations) published. Geographically, most of the countries featured in the top ten publication volumes are situated in Australia, North America, and Europe, with China being the only exception. Notably, all these countries, except China, belong to the group of developed nations, suggesting that economic strength is a prerequisite for fostering research in the digital technology education application field.
This study presents a visual representation of the publication output and cooperation relationships among different countries in the field of digital technology education application research. Specifically, a chord diagram is employed to display the top 30 countries in terms of publication output, as depicted in Fig. 3 . The chord diagram is composed of nodes and chords, where the nodes are positioned as scattered points along the circumference, and the length of each node corresponds to the publication output, with longer lengths indicating higher publication output. The chords, on the other hand, represent the cooperation relationships between any two countries, and are weighted based on the degree of closeness of the cooperation, with wider chords indicating closer cooperation. Through the analysis of the cooperation relationships, the findings suggest that the main publishing countries in this field are engaged in cooperative relationships with each other, indicating a relatively high level of international academic exchange and research internationalization.
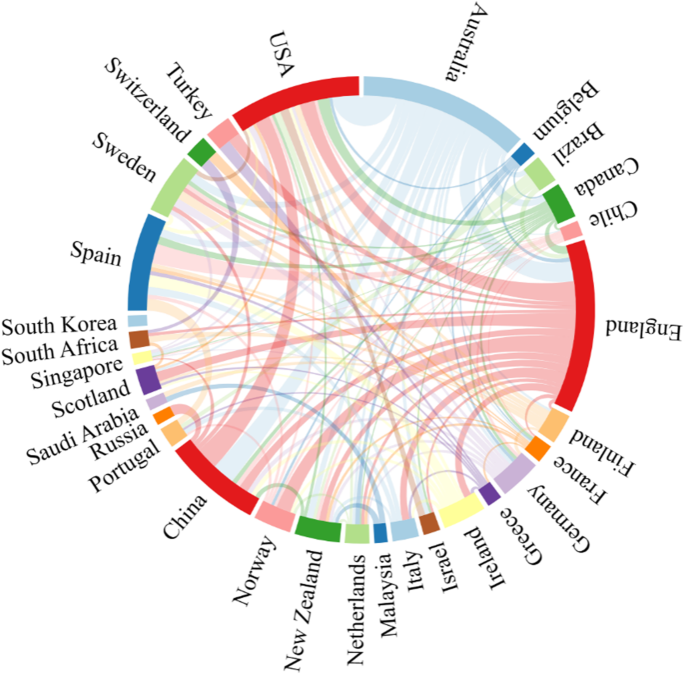
In the diagram, nodes are scattered along the circumference of a circle, with the length of each node representing the volume of publications. The weighted arcs connecting any two points on the circle are known as chords, representing the collaborative relationship between the two, with the width of the arc indicating the closeness of the collaboration.
Further analyzing Fig. 3 , we can extract more valuable information, enabling a deeper understanding of the connections between countries in the research field of digital technology in educational applications. It is evident that certain countries, such as the United States, China, and England, display thicker connections, indicating robust collaborative relationships in terms of productivity. These thicker lines signify substantial mutual contributions and shared objectives in certain sectors or fields, highlighting the interconnectedness and global integration in these areas. By delving deeper, we can also explore potential future collaboration opportunities through the chord diagram, identifying possible partners to propel research and development in this field. In essence, the chord diagram successfully encapsulates and conveys the multi-dimensionality of global productivity and cooperation, allowing for a comprehensive understanding of the intricate inter-country relationships and networks in a global context, providing valuable guidance and insights for future research and collaborations.
An in-depth examination of the publishing institutions is provided in Table 5 , showcasing the foremost 10 institutions ranked by their publication volume. Notably, Monash University and Australian Catholic University, situated in Australia, have recorded the most prolific publications within the digital technology education application realm, with 22 and 10 publications respectively. Moreover, the University of Oslo from Norway is featured among the top 10 publishing institutions, with an impressive average citation count of 64 per publication. It is worth highlighting that six institutions based in the United Kingdom were also ranked within the top 10 publishing institutions, signifying their leading position in this area of research.
Analysis of journals
Journals are the main carriers for publishing high-quality papers. Some scholars point out that the two key factors to measure the influence of journals in the specified field are the number of articles published and the number of citations. The more papers published in a magazine and the more citations, the greater its influence (Dzikowski, 2018 ). Therefore, this study utilized VOSviewer to statistically analyze the top 10 journals with the most publications in the field of digital technology in education and calculated the average citations per article (see Table 6 ).
Based on Table 6 , it is apparent that the highest number of articles in the domain of digital technology in education research were published in Education and Information Technologies (47 articles), Computers & Education (34 articles), and British Journal of Educational Technology (32 articles), indicating a higher article output compared to other journals. This underscores the fact that these three journals concentrate more on the application of digital technology in education. Furthermore, several other journals, such as Technology Pedagogy and Education and Sustainability, have published more than 15 articles in this domain. Sustainability represents the open access movement, which has notably facilitated research progress in this field, indicating that the development of open access journals in recent years has had a significant impact. Although there is still considerable disagreement among scholars on the optimal approach to achieve open access, the notion that research outcomes should be accessible to all is widely recognized (Huang et al. 2020 ). On further analysis of the research fields to which these journals belong, except for Sustainability, it is evident that they all pertain to educational technology, thus providing a qualitative definition of the research area of digital technology education from the perspective of journals.
Temporal keyword analysis: thematic evolution (RQ2)
The evolution of research themes is a dynamic process, and previous studies have attempted to present the developmental trajectory of fields by drawing keyword networks in phases (Kumar et al. 2021 ; Chen et al. 2022b ). To understand the shifts in research topics across different periods, this study follows past research and, based on the significant changes in the research field and corresponding technological advancements during the outlined periods, divides the timeline into four stages (the first stage from January 2000 to December 2005, the second stage from January 2006 to December 2011, the third stage from January 2012 to December 2017; and the fourth stage from January 2018 to December 2022). The division into these four stages was determined through a combination of bibliometric analysis and literature review, which presented a clear trajectory of the field’s development. The research analyzes the keyword networks for each time period (as there are only three articles in the first stage, it was not possible to generate an appropriate keyword co-occurrence map, hence only the keyword co-occurrence maps from the second to the fourth stages are provided), to understand the evolutionary track of the digital technology education application research field over time.
2000.1–2005.12: germination period
From January 2000 to December 2005, digital technology education application research was in its infancy. Only three studies focused on digital technology, all of which were related to computers. Due to the popularity of computers, the home became a new learning environment, highlighting the important role of digital technology in expanding the scope of learning spaces (Sutherland et al. 2000 ). In specific disciplines and contexts, digital technology was first favored in medical clinical practice, becoming an important tool for supporting the learning of clinical knowledge and practice (Tegtmeyer et al. 2001 ; Durfee et al. 2003 ).
2006.1–2011.12: initial development period
Between January 2006 and December 2011, it was the initial development period of digital technology education research. Significant growth was observed in research related to digital technology, and discussions and theoretical analyses about “digital natives” emerged. During this phase, scholars focused on the debate about “how to use digital technology reasonably” and “whether current educational models and school curriculum design need to be adjusted on a large scale” (Bennett and Maton, 2010 ; Selwyn, 2009 ; Margaryan et al. 2011 ). These theoretical and speculative arguments provided a unique perspective on the impact of cognitive digital technology on education and teaching. As can be seen from the vocabulary such as “rethinking”, “disruptive pedagogy”, and “attitude” in Fig. 4 , many scholars joined the calm reflection and analysis under the trend of digital technology (Laurillard, 2008 ; Vratulis et al. 2011 ). During this phase, technology was still undergoing dramatic changes. The development of mobile technology had already caught the attention of many scholars (Wong et al. 2011 ), but digital technology represented by computers was still very active (Selwyn et al. 2011 ). The change in technological form would inevitably lead to educational transformation. Collins and Halverson ( 2010 ) summarized the prospects and challenges of using digital technology for learning and educational practices, believing that digital technology would bring a disruptive revolution to the education field and bring about a new educational system. In addition, the term “teacher education” in Fig. 4 reflects the impact of digital technology development on teachers. The rapid development of technology has widened the generation gap between teachers and students. To ensure smooth communication between teachers and students, teachers must keep up with the trend of technological development and establish a lifelong learning concept (Donnison, 2009 ).
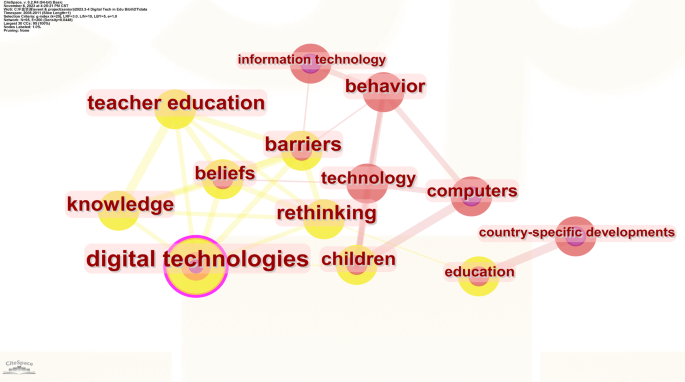
In the diagram, each node represents a keyword, with the size of the node indicating the frequency of occurrence of the keyword. The connections represent the co-occurrence relationships between keywords, with a higher frequency of co-occurrence resulting in tighter connections.
2012.1–2017.12: critical exploration period
During the period spanning January 2012 to December 2017, the application of digital technology in education research underwent a significant exploration phase. As can be seen from Fig. 5 , different from the previous stage, the specific elements of specific digital technology have started to increase significantly, including the enrichment of technological contexts, the greater variety of research methods, and the diversification of learning modes. Moreover, the temporal and spatial dimensions of the learning environment were further de-emphasized, as noted in previous literature (Za et al. 2014 ). Given the rapidly accelerating pace of technological development, the education system in the digital era is in urgent need of collaborative evolution and reconstruction, as argued by Davis, Eickelmann, and Zaka ( 2013 ).
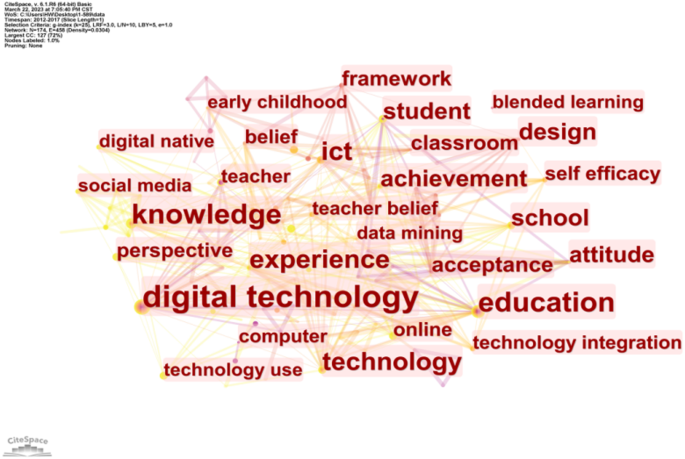
In the domain of digital technology, social media has garnered substantial scholarly attention as a promising avenue for learning, as noted by Pasquini and Evangelopoulos ( 2016 ). The implementation of social media in education presents several benefits, including the liberation of education from the restrictions of physical distance and time, as well as the erasure of conventional educational boundaries. The user-generated content (UGC) model in social media has emerged as a crucial source for knowledge creation and distribution, with the widespread adoption of mobile devices. Moreover, social networks have become an integral component of ubiquitous learning environments (Hwang et al. 2013 ). The utilization of social media allows individuals to function as both knowledge producers and recipients, which leads to a blurring of the conventional roles of learners and teachers. On mobile platforms, the roles of learners and teachers are not fixed, but instead interchangeable.
In terms of research methodology, the prevalence of empirical studies with survey designs in the field of educational technology during this period is evident from the vocabulary used, such as “achievement,” “acceptance,” “attitude,” and “ict.” in Fig. 5 . These studies aim to understand learners’ willingness to adopt and attitudes towards new technologies, and some seek to investigate the impact of digital technologies on learning outcomes through quasi-experimental designs (Domínguez et al. 2013 ). Among these empirical studies, mobile learning emerged as a hot topic, and this is not surprising. First, the advantages of mobile learning environments over traditional ones have been empirically demonstrated (Hwang et al. 2013 ). Second, learners born around the turn of the century have been heavily influenced by digital technologies and have developed their own learning styles that are more open to mobile devices as a means of learning. Consequently, analyzing mobile learning as a relatively novel mode of learning has become an important issue for scholars in the field of educational technology.
The intervention of technology has led to the emergence of several novel learning modes, with the blended learning model being the most representative one in the current phase. Blended learning, a novel concept introduced in the information age, emphasizes the integration of the benefits of traditional learning methods and online learning. This learning mode not only highlights the prominent role of teachers in guiding, inspiring, and monitoring the learning process but also underlines the importance of learners’ initiative, enthusiasm, and creativity in the learning process. Despite being an early conceptualization, blended learning’s meaning has been expanded by the widespread use of mobile technology and social media in education. The implementation of new technologies, particularly mobile devices, has resulted in the transformation of curriculum design and increased flexibility and autonomy in students’ learning processes (Trujillo Maza et al. 2016 ), rekindling scholarly attention to this learning mode. However, some scholars have raised concerns about the potential drawbacks of the blended learning model, such as its significant impact on the traditional teaching system, the lack of systematic coping strategies and relevant policies in several schools and regions (Moskal et al. 2013 ).
2018.1–2022.12: accelerated transformation period
The period spanning from January 2018 to December 2022 witnessed a rapid transformation in the application of digital technology in education research. The field of digital technology education research reached a peak period of publication, largely influenced by factors such as the COVID-19 pandemic (Yu et al. 2023 ). Research during this period was built upon the achievements, attitudes, and social media of the previous phase, and included more elements that reflect the characteristics of this research field, such as digital literacy, digital competence, and professional development, as depicted in Fig. 6 . Alongside this, scholars’ expectations for the value of digital technology have expanded, and the pursuit of improving learning efficiency and performance is no longer the sole focus. Some research now aims to cultivate learners’ motivation and enhance their self-efficacy by applying digital technology in a reasonable manner, as demonstrated by recent studies (Beardsley et al. 2021 ; Creely et al. 2021 ).
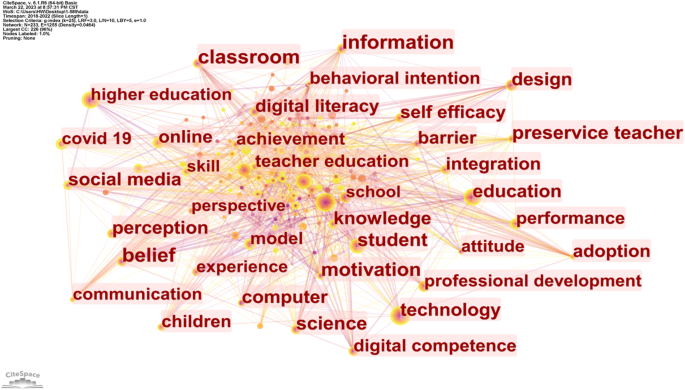
The COVID-19 pandemic has emerged as a crucial backdrop for the digital technology’s role in sustaining global education, as highlighted by recent scholarly research (Zhou et al. 2022 ; Pan and Zhang, 2020 ; Mo et al. 2022 ). The online learning environment, which is supported by digital technology, has become the primary battleground for global education (Yu, 2022 ). This social context has led to various studies being conducted, with some scholars positing that the pandemic has impacted the traditional teaching order while also expanding learning possibilities in terms of patterns and forms (Alabdulaziz, 2021 ). Furthermore, the pandemic has acted as a catalyst for teacher teaching and technological innovation, and this viewpoint has been empirically substantiated (Moorhouse and Wong, 2021 ). Additionally, some scholars believe that the pandemic’s push is a crucial driving force for the digital transformation of the education system, serving as an essential mechanism for overcoming the system’s inertia (Romero et al. 2021 ).
The rapid outbreak of the pandemic posed a challenge to the large-scale implementation of digital technologies, which was influenced by a complex interplay of subjective and objective factors. Objective constraints included the lack of infrastructure in some regions to support digital technologies, while subjective obstacles included psychological resistance among certain students and teachers (Moorhouse, 2021 ). These factors greatly impacted the progress of online learning during the pandemic. Additionally, Timotheou et al. ( 2023 ) conducted a comprehensive systematic review of existing research on digital technology use during the pandemic, highlighting the critical role played by various factors such as learners’ and teachers’ digital skills, teachers’ personal attributes and professional development, school leadership and management, and administration in facilitating the digitalization and transformation of schools.
The current stage of research is characterized by the pivotal term “digital literacy,” denoting a growing interest in learners’ attitudes and adoption of emerging technologies. Initially, the term “literacy” was restricted to fundamental abilities and knowledge associated with books and print materials (McMillan, 1996 ). However, with the swift advancement of computers and digital technology, there have been various attempts to broaden the scope of literacy beyond its traditional meaning, including game literacy (Buckingham and Burn, 2007 ), information literacy (Eisenberg, 2008 ), and media literacy (Turin and Friesem, 2020 ). Similarly, digital literacy has emerged as a crucial concept, and Gilster and Glister ( 1997 ) were the first to introduce this concept, referring to the proficiency in utilizing technology and processing digital information in academic, professional, and daily life settings. In practical educational settings, learners who possess higher digital literacy often exhibit an aptitude for quickly mastering digital devices and applying them intelligently to education and teaching (Yu, 2022 ).
The utilization of digital technology in education has undergone significant changes over the past two decades, and has been a crucial driver of educational reform with each new technological revolution. The impact of these changes on the underlying logic of digital technology education applications has been noticeable. From computer technology to more recent developments such as virtual reality (VR), augmented reality (AR), and artificial intelligence (AI), the acceleration in digital technology development has been ongoing. Educational reforms spurred by digital technology development continue to be dynamic, as each new digital innovation presents new possibilities and models for teaching practice. This is especially relevant in the post-pandemic era, where the importance of technological progress in supporting teaching cannot be overstated (Mughal et al. 2022 ). Existing digital technologies have already greatly expanded the dimensions of education in both time and space, while future digital technologies aim to expand learners’ perceptions. Researchers have highlighted the potential of integrated technology and immersive technology in the development of the educational metaverse, which is highly anticipated to create a new dimension for the teaching and learning environment, foster a new value system for the discipline of educational technology, and more effectively and efficiently achieve the grand educational blueprint of the United Nations’ Sustainable Development Goals (Zhang et al. 2022 ; Li and Yu, 2023 ).
Hotspot evolution analysis (RQ3)
The examination of keyword evolution reveals a consistent trend in the advancement of digital technology education application research. The emergence and transformation of keywords serve as indicators of the varying research interests in this field. Thus, the utilization of the burst detection function available in CiteSpace allowed for the identification of the top 10 burst words that exhibited a high level of burst strength. This outcome is illustrated in Table 7 .
According to the results presented in Table 7 , the explosive terminology within the realm of digital technology education research has exhibited a concentration mainly between the years 2018 and 2022. Prior to this time frame, the emerging keywords were limited to “information technology” and “computer”. Notably, among them, computer, as an emergent keyword, has always had a high explosive intensity from 2008 to 2018, which reflects the important position of computer in digital technology and is the main carrier of many digital technologies such as Learning Management Systems (LMS) and Assessment and Feedback systems (Barlovits et al. 2022 ).
Since 2018, an increasing number of research studies have focused on evaluating the capabilities of learners to accept, apply, and comprehend digital technologies. As indicated by the use of terms such as “digital literacy” and “digital skill,” the assessment of learners’ digital literacy has become a critical task. Scholarly efforts have been directed towards the development of literacy assessment tools and the implementation of empirical assessments. Furthermore, enhancing the digital literacy of both learners and educators has garnered significant attention. (Nagle, 2018 ; Yu, 2022 ). Simultaneously, given the widespread use of various digital technologies in different formal and informal learning settings, promoting learners’ digital skills has become a crucial objective for contemporary schools (Nygren et al. 2019 ; Forde and OBrien, 2022 ).
Since 2020, the field of applied research on digital technology education has witnessed the emergence of three new hotspots, all of which have been affected to some extent by the pandemic. Firstly, digital technology has been widely applied in physical education, which is one of the subjects that has been severely affected by the pandemic (Parris et al. 2022 ; Jiang and Ning, 2022 ). Secondly, digital transformation has become an important measure for most schools, especially higher education institutions, to cope with the impact of the pandemic globally (García-Morales et al. 2021 ). Although the concept of digital transformation was proposed earlier, the COVID-19 pandemic has greatly accelerated this transformation process. Educational institutions must carefully redesign their educational products to face this new situation, providing timely digital learning methods, environments, tools, and support systems that have far-reaching impacts on modern society (Krishnamurthy, 2020 ; Salas-Pilco et al. 2022 ). Moreover, the professional development of teachers has become a key mission of educational institutions in the post-pandemic era. Teachers need to have a certain level of digital literacy and be familiar with the tools and online teaching resources used in online teaching, which has become a research hotspot today. Organizing digital skills training for teachers to cope with the application of emerging technologies in education is an important issue for teacher professional development and lifelong learning (Garzón-Artacho et al. 2021 ). As the main organizers and practitioners of emergency remote teaching (ERT) during the pandemic, teachers must put cognitive effort into their professional development to ensure effective implementation of ERT (Romero-Hall and Jaramillo Cherrez, 2022 ).
The burst word “digital transformation” reveals that we are in the midst of an ongoing digital technology revolution. With the emergence of innovative digital technologies such as ChatGPT and Microsoft 365 Copilot, technology trends will continue to evolve, albeit unpredictably. While the impact of these advancements on school education remains uncertain, it is anticipated that the widespread integration of technology will significantly affect the current education system. Rejecting emerging technologies without careful consideration is unwise. Like any revolution, the technological revolution in the education field has both positive and negative aspects. Detractors argue that digital technology disrupts learning and memory (Baron, 2021 ) or causes learners to become addicted and distracted from learning (Selwyn and Aagaard, 2020 ). On the other hand, the prudent use of digital technology in education offers a glimpse of a golden age of open learning. Educational leaders and practitioners have the opportunity to leverage cutting-edge digital technologies to address current educational challenges and develop a rational path for the sustainable and healthy growth of education.
Discussion on performance analysis (RQ1)
The field of digital technology education application research has experienced substantial growth since the turn of the century, a phenomenon that is quantifiably apparent through an analysis of authorship, country/region contributions, and institutional engagement. This expansion reflects the increased integration of digital technologies in educational settings and the heightened scholarly interest in understanding and optimizing their use.
Discussion on authorship productivity in digital technology education research
The authorship distribution within digital technology education research is indicative of the field’s intellectual structure and depth. A primary figure in this domain is Neil Selwyn, whose substantial citation rate underscores the profound impact of his work. His focus on the implications of digital technology in higher education and educational sociology has proven to be seminal. Selwyn’s research trajectory, especially the exploration of spatiotemporal extensions of education through technology, provides valuable insights into the multifaceted role of digital tools in learning processes (Selwyn et al. 2019 ).
Other notable contributors, like Henderson and Edwards, present diversified research interests, such as the impact of digital technologies during the pandemic and their application in early childhood education, respectively. Their varied focuses highlight the breadth of digital technology education research, encompassing pedagogical innovation, technological adaptation, and policy development.
Discussion on country/region-level productivity and collaboration
At the country/region level, the United Kingdom, specifically England, emerges as a leading contributor with 92 published papers and a significant citation count. This is closely followed by Australia and the United States, indicating a strong English-speaking research axis. Such geographical concentration of scholarly output often correlates with investment in research and development, technological infrastructure, and the prevalence of higher education institutions engaging in cutting-edge research.
China’s notable inclusion as the only non-Western country among the top contributors to the field suggests a growing research capacity and interest in digital technology in education. However, the lower average citation per paper for China could reflect emerging engagement or different research focuses that may not yet have achieved the same international recognition as Western counterparts.
The chord diagram analysis furthers this understanding, revealing dense interconnections between countries like the United States, China, and England, which indicates robust collaborations. Such collaborations are fundamental in addressing global educational challenges and shaping international research agendas.
Discussion on institutional-level contributions to digital technology education
Institutional productivity in digital technology education research reveals a constellation of universities driving the field forward. Monash University and the Australian Catholic University have the highest publication output, signaling Australia’s significant role in advancing digital education research. The University of Oslo’s remarkable average citation count per publication indicates influential research contributions, potentially reflecting high-quality studies that resonate with the broader academic community.
The strong showing of UK institutions, including the University of London, The Open University, and the University of Cambridge, reinforces the UK’s prominence in this research field. Such institutions are often at the forefront of pedagogical innovation, benefiting from established research cultures and funding mechanisms that support sustained inquiry into digital education.
Discussion on journal publication analysis
An examination of journal outputs offers a lens into the communicative channels of the field’s knowledge base. Journals such as Education and Information Technologies , Computers & Education , and the British Journal of Educational Technology not only serve as the primary disseminators of research findings but also as indicators of research quality and relevance. The impact factor (IF) serves as a proxy for the quality and influence of these journals within the academic community.
The high citation counts for articles published in Computers & Education suggest that research disseminated through this medium has a wide-reaching impact and is of particular interest to the field. This is further evidenced by its significant IF of 11.182, indicating that the journal is a pivotal platform for seminal work in the application of digital technology in education.
The authorship, regional, and institutional productivity in the field of digital technology education application research collectively narrate the evolution of this domain since the turn of the century. The prominence of certain authors and countries underscores the importance of socioeconomic factors and existing academic infrastructure in fostering research productivity. Meanwhile, the centrality of specific journals as outlets for high-impact research emphasizes the role of academic publishing in shaping the research landscape.
As the field continues to grow, future research may benefit from leveraging the collaborative networks that have been elucidated through this analysis, perhaps focusing on underrepresented regions to broaden the scope and diversity of research. Furthermore, the stabilization of publication numbers in recent years invites a deeper exploration into potential plateaus in research trends or saturation in certain sub-fields, signaling an opportunity for novel inquiries and methodological innovations.
Discussion on the evolutionary trends (RQ2)
The evolution of the research field concerning the application of digital technology in education over the past two decades is a story of convergence, diversification, and transformation, shaped by rapid technological advancements and shifting educational paradigms.
At the turn of the century, the inception of digital technology in education was largely exploratory, with a focus on how emerging computer technologies could be harnessed to enhance traditional learning environments. Research from this early period was primarily descriptive, reflecting on the potential and challenges of incorporating digital tools into the educational setting. This phase was critical in establishing the fundamental discourse that would guide subsequent research, as it set the stage for understanding the scope and impact of digital technology in learning spaces (Wang et al. 2023 ).
As the first decade progressed, the narrative expanded to encompass the pedagogical implications of digital technologies. This was a period of conceptual debates, where terms like “digital natives” and “disruptive pedagogy” entered the academic lexicon, underscoring the growing acknowledgment of digital technology as a transformative force within education (Bennett and Maton, 2010 ). During this time, the research began to reflect a more nuanced understanding of the integration of technology, considering not only its potential to change where and how learning occurred but also its implications for educational equity and access.
In the second decade, with the maturation of internet connectivity and mobile technology, the focus of research shifted from theoretical speculations to empirical investigations. The proliferation of digital devices and the ubiquity of social media influenced how learners interacted with information and each other, prompting a surge in studies that sought to measure the impact of these tools on learning outcomes. The digital divide and issues related to digital literacy became central concerns, as scholars explored the varying capacities of students and educators to engage with technology effectively.
Throughout this period, there was an increasing emphasis on the individualization of learning experiences, facilitated by adaptive technologies that could cater to the unique needs and pacing of learners (Jing et al. 2023a ). This individualization was coupled with a growing recognition of the importance of collaborative learning, both online and offline, and the role of digital tools in supporting these processes. Blended learning models, which combined face-to-face instruction with online resources, emerged as a significant trend, advocating for a balance between traditional pedagogies and innovative digital strategies.
The later years, particularly marked by the COVID-19 pandemic, accelerated the necessity for digital technology in education, transforming it from a supplementary tool to an essential platform for delivering education globally (Mo et al. 2022 ; Mustapha et al. 2021 ). This era brought about an unprecedented focus on online learning environments, distance education, and virtual classrooms. Research became more granular, examining not just the pedagogical effectiveness of digital tools, but also their role in maintaining continuity of education during crises, their impact on teacher and student well-being, and their implications for the future of educational policy and infrastructure.
Across these two decades, the research field has seen a shift from examining digital technology as an external addition to the educational process, to viewing it as an integral component of curriculum design, instructional strategies, and even assessment methods. The emergent themes have broadened from a narrow focus on specific tools or platforms to include wider considerations such as data privacy, ethical use of technology, and the environmental impact of digital tools.
Moreover, the field has moved from considering the application of digital technology in education as a primarily cognitive endeavor to recognizing its role in facilitating socio-emotional learning, digital citizenship, and global competencies. Researchers have increasingly turned their attention to the ways in which technology can support collaborative skills, cultural understanding, and ethical reasoning within diverse student populations.
In summary, the past over twenty years in the research field of digital technology applications in education have been characterized by a progression from foundational inquiries to complex analyses of digital integration. This evolution has mirrored the trajectory of technology itself, from a facilitative tool to a pervasive ecosystem defining contemporary educational experiences. As we look to the future, the field is poised to delve into the implications of emerging technologies like AI, AR, and VR, and their potential to redefine the educational landscape even further. This ongoing metamorphosis suggests that the application of digital technology in education will continue to be a rich area of inquiry, demanding continual adaptation and forward-thinking from educators and researchers alike.
Discussion on the study of research hotspots (RQ3)
The analysis of keyword evolution in digital technology education application research elucidates the current frontiers in the field, reflecting a trajectory that is in tandem with the rapidly advancing digital age. This landscape is sculpted by emergent technological innovations and shaped by the demands of an increasingly digital society.
Interdisciplinary integration and pedagogical transformation
One of the frontiers identified from recent keyword bursts includes the integration of digital technology into diverse educational contexts, particularly noted with the keyword “physical education.” The digitalization of disciplines traditionally characterized by physical presence illustrates the pervasive reach of technology and signifies a push towards interdisciplinary integration where technology is not only a facilitator but also a transformative agent. This integration challenges educators to reconceptualize curriculum delivery to accommodate digital tools that can enhance or simulate the physical aspects of learning.
Digital literacy and skills acquisition
Another pivotal frontier is the focus on “digital literacy” and “digital skill”, which has intensified in recent years. This suggests a shift from mere access to technology towards a comprehensive understanding and utilization of digital tools. In this realm, the emphasis is not only on the ability to use technology but also on critical thinking, problem-solving, and the ethical use of digital resources (Yu, 2022 ). The acquisition of digital literacy is no longer an additive skill but a fundamental aspect of modern education, essential for navigating and contributing to the digital world.
Educational digital transformation
The keyword “digital transformation” marks a significant research frontier, emphasizing the systemic changes that education institutions must undergo to align with the digital era (Romero et al. 2021 ). This transformation includes the redesigning of learning environments, pedagogical strategies, and assessment methods to harness digital technology’s full potential. Research in this area explores the complexity of institutional change, addressing the infrastructural, cultural, and policy adjustments needed for a seamless digital transition.
Engagement and participation
Further exploration into “engagement” and “participation” underscores the importance of student-centered learning environments that are mediated by technology. The current frontiers examine how digital platforms can foster collaboration, inclusivity, and active learning, potentially leading to more meaningful and personalized educational experiences. Here, the use of technology seeks to support the emotional and cognitive aspects of learning, moving beyond the transactional view of education to one that is relational and interactive.
Professional development and teacher readiness
As the field evolves, “professional development” emerges as a crucial area, particularly in light of the pandemic which necessitated emergency remote teaching. The need for teacher readiness in a digital age is a pressing frontier, with research focusing on the competencies required for educators to effectively integrate technology into their teaching practices. This includes familiarity with digital tools, pedagogical innovation, and an ongoing commitment to personal and professional growth in the digital domain.
Pandemic as a catalyst
The recent pandemic has acted as a catalyst for accelerated research and application in this field, particularly in the domains of “digital transformation,” “professional development,” and “physical education.” This period has been a litmus test for the resilience and adaptability of educational systems to continue their operations in an emergency. Research has thus been directed at understanding how digital technologies can support not only continuity but also enhance the quality and reach of education in such contexts.
Ethical and societal considerations
The frontier of digital technology in education is also expanding to consider broader ethical and societal implications. This includes issues of digital equity, data privacy, and the sociocultural impact of technology on learning communities. The research explores how educational technology can be leveraged to address inequities and create more equitable learning opportunities for all students, regardless of their socioeconomic background.
Innovation and emerging technologies
Looking forward, the frontiers are set to be influenced by ongoing and future technological innovations, such as artificial intelligence (AI) (Wu and Yu, 2023 ; Chen et al. 2022a ). The exploration into how these technologies can be integrated into educational practices to create immersive and adaptive learning experiences represents a bold new chapter for the field.
In conclusion, the current frontiers of research on the application of digital technology in education are multifaceted and dynamic. They reflect an overarching movement towards deeper integration of technology in educational systems and pedagogical practices, where the goals are not only to facilitate learning but to redefine it. As these frontiers continue to expand and evolve, they will shape the educational landscape, requiring a concerted effort from researchers, educators, policymakers, and technologists to navigate the challenges and harness the opportunities presented by the digital revolution in education.
Conclusions and future research
Conclusions.
The utilization of digital technology in education is a research area that cuts across multiple technical and educational domains and continues to experience dynamic growth due to the continuous progress of technology. In this study, a systematic review of this field was conducted through bibliometric techniques to examine its development trajectory. The primary focus of the review was to investigate the leading contributors, productive national institutions, significant publications, and evolving development patterns. The study’s quantitative analysis resulted in several key conclusions that shed light on this research field’s current state and future prospects.
(1) The research field of digital technology education applications has entered a stage of rapid development, particularly in recent years due to the impact of the pandemic, resulting in a peak of publications. Within this field, several key authors (Selwyn, Henderson, Edwards, etc.) and countries/regions (England, Australia, USA, etc.) have emerged, who have made significant contributions. International exchanges in this field have become frequent, with a high degree of internationalization in academic research. Higher education institutions in the UK and Australia are the core productive forces in this field at the institutional level.
(2) Education and Information Technologies , Computers & Education , and the British Journal of Educational Technology are notable journals that publish research related to digital technology education applications. These journals are affiliated with the research field of educational technology and provide effective communication platforms for sharing digital technology education applications.
(3) Over the past two decades, research on digital technology education applications has progressed from its early stages of budding, initial development, and critical exploration to accelerated transformation, and it is currently approaching maturity. Technological progress and changes in the times have been key driving forces for educational transformation and innovation, and both have played important roles in promoting the continuous development of education.
(4) Influenced by the pandemic, three emerging frontiers have emerged in current research on digital technology education applications, which are physical education, digital transformation, and professional development under the promotion of digital technology. These frontier research hotspots reflect the core issues that the education system faces when encountering new technologies. The evolution of research hotspots shows that technology breakthroughs in education’s original boundaries of time and space create new challenges. The continuous self-renewal of education is achieved by solving one hotspot problem after another.
The present study offers significant practical implications for scholars and practitioners in the field of digital technology education applications. Firstly, it presents a well-defined framework of the existing research in this area, serving as a comprehensive guide for new entrants to the field and shedding light on the developmental trajectory of this research domain. Secondly, the study identifies several contemporary research hotspots, thus offering a valuable decision-making resource for scholars aiming to explore potential research directions. Thirdly, the study undertakes an exhaustive analysis of published literature to identify core journals in the field of digital technology education applications, with Sustainability being identified as a promising open access journal that publishes extensively on this topic. This finding can potentially facilitate scholars in selecting appropriate journals for their research outputs.
Limitation and future research
Influenced by some objective factors, this study also has some limitations. First of all, the bibliometrics analysis software has high standards for data. In order to ensure the quality and integrity of the collected data, the research only selects the periodical papers in SCIE and SSCI indexes, which are the core collection of Web of Science database, and excludes other databases, conference papers, editorials and other publications, which may ignore some scientific research and original opinions in the field of digital technology education and application research. In addition, although this study used professional software to carry out bibliometric analysis and obtained more objective quantitative data, the analysis and interpretation of data will inevitably have a certain subjective color, and the influence of subjectivity on data analysis cannot be completely avoided. As such, future research endeavors will broaden the scope of literature screening and proactively engage scholars in the field to gain objective and state-of-the-art insights, while minimizing the adverse impact of personal subjectivity on research analysis.
Data availability
The datasets analyzed during the current study are available in the Dataverse repository: https://doi.org/10.7910/DVN/F9QMHY
Alabdulaziz MS (2021) COVID-19 and the use of digital technology in mathematics education. Educ Inf Technol 26(6):7609–7633. https://doi.org/10.1007/s10639-021-10602-3
Arif TB, Munaf U, Ul-Haque I (2023) The future of medical education and research: is ChatGPT a blessing or blight in disguise? Med Educ Online 28. https://doi.org/10.1080/10872981.2023.2181052
Banerjee M, Chiew D, Patel KT, Johns I, Chappell D, Linton N, Cole GD, Francis DP, Szram J, Ross J, Zaman S (2021) The impact of artificial intelligence on clinical education: perceptions of postgraduate trainee doctors in London (UK) and recommendations for trainers. BMC Med Educ 21. https://doi.org/10.1186/s12909-021-02870-x
Barlovits S, Caldeira A, Fesakis G, Jablonski S, Koutsomanoli Filippaki D, Lázaro C, Ludwig M, Mammana MF, Moura A, Oehler DXK, Recio T, Taranto E, Volika S(2022) Adaptive, synchronous, and mobile online education: developing the ASYMPTOTE learning environment. Mathematics 10:1628. https://doi.org/10.3390/math10101628
Article Google Scholar
Baron NS(2021) Know what? How digital technologies undermine learning and remembering J Pragmat 175:27–37. https://doi.org/10.1016/j.pragma.2021.01.011
Batista J, Morais NS, Ramos F (2016) Researching the use of communication technologies in higher education institutions in Portugal. https://doi.org/10.4018/978-1-5225-0571-6.ch057
Beardsley M, Albó L, Aragón P, Hernández-Leo D (2021) Emergency education effects on teacher abilities and motivation to use digital technologies. Br J Educ Technol 52. https://doi.org/10.1111/bjet.13101
Bennett S, Maton K(2010) Beyond the “digital natives” debate: towards a more nuanced understanding of students’ technology experiences J Comput Assist Learn 26:321–331. https://doi.org/10.1111/j.1365-2729.2010.00360.x
Buckingham D, Burn A (2007) Game literacy in theory and practice 16:323–349
Google Scholar
Bulfin S, Pangrazio L, Selwyn N (2014) Making “MOOCs”: the construction of a new digital higher education within news media discourse. In: The International Review of Research in Open and Distributed Learning 15. https://doi.org/10.19173/irrodl.v15i5.1856
Camilleri MA, Camilleri AC(2016) Digital learning resources and ubiquitous technologies in education Technol Knowl Learn 22:65–82. https://doi.org/10.1007/s10758-016-9287-7
Chen C(2006) CiteSpace II: detecting and visualizing emerging trends and transient patterns in scientific literature J Am Soc Inf Sci Technol 57:359–377. https://doi.org/10.1002/asi.20317
Chen J, Dai J, Zhu K, Xu L(2022) Effects of extended reality on language learning: a meta-analysis Front Psychol 13:1016519. https://doi.org/10.3389/fpsyg.2022.1016519
Article PubMed PubMed Central Google Scholar
Chen J, Wang CL, Tang Y (2022b) Knowledge mapping of volunteer motivation: a bibliometric analysis and cross-cultural comparative study. Front Psychol 13. https://doi.org/10.3389/fpsyg.2022.883150
Cohen A, Soffer T, Henderson M(2022) Students’ use of technology and their perceptions of its usefulness in higher education: International comparison J Comput Assist Learn 38(5):1321–1331. https://doi.org/10.1111/jcal.12678
Collins A, Halverson R(2010) The second educational revolution: rethinking education in the age of technology J Comput Assist Learn 26:18–27. https://doi.org/10.1111/j.1365-2729.2009.00339.x
Conole G, Alevizou P (2010) A literature review of the use of Web 2.0 tools in higher education. Walton Hall, Milton Keynes, UK: the Open University, retrieved 17 February
Creely E, Henriksen D, Crawford R, Henderson M(2021) Exploring creative risk-taking and productive failure in classroom practice. A case study of the perceived self-efficacy and agency of teachers at one school Think Ski Creat 42:100951. https://doi.org/10.1016/j.tsc.2021.100951
Davis N, Eickelmann B, Zaka P(2013) Restructuring of educational systems in the digital age from a co-evolutionary perspective J Comput Assist Learn 29:438–450. https://doi.org/10.1111/jcal.12032
De Belli N (2009) Bibliometrics and citation analysis: from the science citation index to cybermetrics, Scarecrow Press. https://doi.org/10.1111/jcal.12032
Domínguez A, Saenz-de-Navarrete J, de-Marcos L, Fernández-Sanz L, Pagés C, Martínez-Herráiz JJ(2013) Gamifying learning experiences: practical implications and outcomes Comput Educ 63:380–392. https://doi.org/10.1016/j.compedu.2012.12.020
Donnison S (2009) Discourses in conflict: the relationship between Gen Y pre-service teachers, digital technologies and lifelong learning. Australasian J Educ Technol 25. https://doi.org/10.14742/ajet.1138
Durfee SM, Jain S, Shaffer K (2003) Incorporating electronic media into medical student education. Acad Radiol 10:205–210. https://doi.org/10.1016/s1076-6332(03)80046-6
Dzikowski P(2018) A bibliometric analysis of born global firms J Bus Res 85:281–294. https://doi.org/10.1016/j.jbusres.2017.12.054
van Eck NJ, Waltman L(2009) Software survey: VOSviewer, a computer program for bibliometric mapping Scientometrics 84:523–538 https://doi.org/10.1007/s11192-009-0146-3
Edwards S(2013) Digital play in the early years: a contextual response to the problem of integrating technologies and play-based pedagogies in the early childhood curriculum Eur Early Child Educ Res J 21:199–212. https://doi.org/10.1080/1350293x.2013.789190
Edwards S(2015) New concepts of play and the problem of technology, digital media and popular-culture integration with play-based learning in early childhood education Technol Pedagogy Educ 25:513–532 https://doi.org/10.1080/1475939x.2015.1108929
Article MathSciNet Google Scholar
Eisenberg MB(2008) Information literacy: essential skills for the information age DESIDOC J Libr Inf Technol 28:39–47. https://doi.org/10.14429/djlit.28.2.166
Forde C, OBrien A (2022) A literature review of barriers and opportunities presented by digitally enhanced practical skill teaching and learning in health science education. Med Educ Online 27. https://doi.org/10.1080/10872981.2022.2068210
García-Morales VJ, Garrido-Moreno A, Martín-Rojas R (2021) The transformation of higher education after the COVID disruption: emerging challenges in an online learning scenario. Front Psychol 12. https://doi.org/10.3389/fpsyg.2021.616059
Garfield E(2006) The history and meaning of the journal impact factor JAMA 295:90. https://doi.org/10.1001/jama.295.1.90
Article PubMed Google Scholar
Garzón-Artacho E, Sola-Martínez T, Romero-Rodríguez JM, Gómez-García G(2021) Teachers’ perceptions of digital competence at the lifelong learning stage Heliyon 7:e07513. https://doi.org/10.1016/j.heliyon.2021.e07513
Gaviria-Marin M, Merigó JM, Baier-Fuentes H(2019) Knowledge management: a global examination based on bibliometric analysis Technol Forecast Soc Change 140:194–220. https://doi.org/10.1016/j.techfore.2018.07.006
Gilster P, Glister P (1997) Digital literacy. Wiley Computer Pub, New York
Greenhow C, Lewin C(2015) Social media and education: reconceptualizing the boundaries of formal and informal learning Learn Media Technol 41:6–30. https://doi.org/10.1080/17439884.2015.1064954
Hawkins DT(2001) Bibliometrics of electronic journals in information science Infor Res 7(1):7–1. http://informationr.net/ir/7-1/paper120.html
Henderson M, Selwyn N, Finger G, Aston R(2015) Students’ everyday engagement with digital technology in university: exploring patterns of use and “usefulness J High Educ Policy Manag 37:308–319 https://doi.org/10.1080/1360080x.2015.1034424
Huang CK, Neylon C, Hosking R, Montgomery L, Wilson KS, Ozaygen A, Brookes-Kenworthy C (2020) Evaluating the impact of open access policies on research institutions. eLife 9. https://doi.org/10.7554/elife.57067
Hwang GJ, Tsai CC(2011) Research trends in mobile and ubiquitous learning: a review of publications in selected journals from 2001 to 2010 Br J Educ Technol 42:E65–E70. https://doi.org/10.1111/j.1467-8535.2011.01183.x
Hwang GJ, Wu PH, Zhuang YY, Huang YM(2013) Effects of the inquiry-based mobile learning model on the cognitive load and learning achievement of students Interact Learn Environ 21:338–354. https://doi.org/10.1080/10494820.2011.575789
Jiang S, Ning CF (2022) Interactive communication in the process of physical education: are social media contributing to the improvement of physical training performance. Universal Access Inf Soc, 1–10. https://doi.org/10.1007/s10209-022-00911-w
Jing Y, Zhao L, Zhu KK, Wang H, Wang CL, Xia Q(2023) Research landscape of adaptive learning in education: a bibliometric study on research publications from 2000 to 2022 Sustainability 15:3115–3115. https://doi.org/10.3390/su15043115
Jing Y, Wang CL, Chen Y, Wang H, Yu T, Shadiev R (2023b) Bibliometric mapping techniques in educational technology research: a systematic literature review. Educ Inf Technol 1–29. https://doi.org/10.1007/s10639-023-12178-6
Krishnamurthy S (2020) The future of business education: a commentary in the shadow of the Covid-19 pandemic. J Bus Res. https://doi.org/10.1016/j.jbusres.2020.05.034
Kumar S, Lim WM, Pandey N, Christopher Westland J (2021) 20 years of electronic commerce research. Electron Commer Res 21:1–40
Kyza EA, Georgiou Y(2018) Scaffolding augmented reality inquiry learning: the design and investigation of the TraceReaders location-based, augmented reality platform Interact Learn Environ 27:211–225. https://doi.org/10.1080/10494820.2018.1458039
Laurillard D(2008) Technology enhanced learning as a tool for pedagogical innovation J Philos Educ 42:521–533. https://doi.org/10.1111/j.1467-9752.2008.00658.x
Li M, Yu Z (2023) A systematic review on the metaverse-based blended English learning. Front Psychol 13. https://doi.org/10.3389/fpsyg.2022.1087508
Luo H, Li G, Feng Q, Yang Y, Zuo M (2021) Virtual reality in K-12 and higher education: a systematic review of the literature from 2000 to 2019. J Comput Assist Learn. https://doi.org/10.1111/jcal.12538
Margaryan A, Littlejohn A, Vojt G(2011) Are digital natives a myth or reality? University students’ use of digital technologies Comput Educ 56:429–440. https://doi.org/10.1016/j.compedu.2010.09.004
McMillan S(1996) Literacy and computer literacy: definitions and comparisons Comput Educ 27:161–170. https://doi.org/10.1016/s0360-1315(96)00026-7
Mo CY, Wang CL, Dai J, Jin P (2022) Video playback speed influence on learning effect from the perspective of personalized adaptive learning: a study based on cognitive load theory. Front Psychology 13. https://doi.org/10.3389/fpsyg.2022.839982
Moorhouse BL (2021) Beginning teaching during COVID-19: newly qualified Hong Kong teachers’ preparedness for online teaching. Educ Stud 1–17. https://doi.org/10.1080/03055698.2021.1964939
Moorhouse BL, Wong KM (2021) The COVID-19 Pandemic as a catalyst for teacher pedagogical and technological innovation and development: teachers’ perspectives. Asia Pac J Educ 1–16. https://doi.org/10.1080/02188791.2021.1988511
Moskal P, Dziuban C, Hartman J (2013) Blended learning: a dangerous idea? Internet High Educ 18:15–23
Mughal MY, Andleeb N, Khurram AFA, Ali MY, Aslam MS, Saleem MN (2022) Perceptions of teaching-learning force about Metaverse for education: a qualitative study. J. Positive School Psychol 6:1738–1745
Mustapha I, Thuy Van N, Shahverdi M, Qureshi MI, Khan N (2021) Effectiveness of digital technology in education during COVID-19 pandemic. a bibliometric analysis. Int J Interact Mob Technol 15:136
Nagle J (2018) Twitter, cyber-violence, and the need for a critical social media literacy in teacher education: a review of the literature. Teach Teach Education 76:86–94
Nazare J, Woolf A, Sysoev I, Ballinger S, Saveski M, Walker M, Roy D (2022) Technology-assisted coaching can increase engagement with learning technology at home and caregivers’ awareness of it. Comput Educ 188:104565
Nguyen UP, Hallinger P (2020) Assessing the distinctive contributions of simulation & gaming to the literature, 1970-2019: a bibliometric review. Simul Gaming 104687812094156. https://doi.org/10.1177/1046878120941569
Nygren H, Nissinen K, Hämäläinen R, Wever B(2019) Lifelong learning: formal, non-formal and informal learning in the context of the use of problem-solving skills in technology-rich environments Br J Educ Technol 50:1759–1770. https://doi.org/10.1111/bjet.12807
Page MJ, McKenzie JE, Bossuyt PM, Boutron I, Hoffmann TC, Mulrow CD, Moher D (2021) The PRISMA 2020 statement: an updated guideline for reporting systematic reviews. Int J Surg 88:105906
Pan SL, Zhang S(2020) From fighting COVID-19 pandemic to tackling sustainable development goals: an opportunity for responsible information systems research Int J Inf Manage 55:102196. https://doi.org/10.1016/j.ijinfomgt.2020.102196
Pan X, Yan E, Cui M, Hua W(2018) Examining the usage, citation, and diffusion patterns of bibliometric mapping software: a comparative study of three tools J Informetr 12:481–493. https://doi.org/10.1016/j.joi.2018.03.005
Parris Z, Cale L, Harris J, Casey A (2022) Physical activity for health, covid-19 and social media: what, where and why?. Movimento, 28. https://doi.org/10.22456/1982-8918.122533
Pasquini LA, Evangelopoulos N (2016) Sociotechnical stewardship in higher education: a field study of social media policy documents. J Comput High Educ 29:218–239
Pérez-Sanagustín M, Hernández-Leo D, Santos P, Delgado Kloos C, Blat J(2014) Augmenting reality and formality of informal and non-formal settings to enhance blended learning IEEE Trans Learn Technol 7:118–131. https://doi.org/10.1109/TLT.2014.2312719
Pinto M, Leite C (2020) Digital technologies in support of students learning in Higher Education: literature review. Digital Education Review 343–360. https://doi.org/10.1344/der.2020.37.343-360
Pires F, Masanet MJ, Tomasena JM, Scolari CA(2022) Learning with YouTube: beyond formal and informal through new actors, strategies and affordances Convergence 28(3):838–853. https://doi.org/10.1177/1354856521102054
Pritchard A (1969) Statistical bibliography or bibliometrics 25:348
Romero M, Romeu T, Guitert M, Baztán P (2021) Digital transformation in higher education: the UOC case. In ICERI2021 Proceedings (pp. 6695–6703). IATED https://doi.org/10.21125/iceri.2021.1512
Romero-Hall E, Jaramillo Cherrez N (2022) Teaching in times of disruption: faculty digital literacy in higher education during the COVID-19 pandemic. Innovations in Education and Teaching International 1–11. https://doi.org/10.1080/14703297.2022.2030782
Rospigliosi PA(2023) Artificial intelligence in teaching and learning: what questions should we ask of ChatGPT? Interactive Learning Environments 31:1–3. https://doi.org/10.1080/10494820.2023.2180191
Salas-Pilco SZ, Yang Y, Zhang Z(2022) Student engagement in online learning in Latin American higher education during the COVID-19 pandemic: a systematic review. Br J Educ Technol 53(3):593–619. https://doi.org/10.1111/bjet.13190
Selwyn N(2009) The digital native-myth and reality In Aslib proceedings 61(4):364–379. https://doi.org/10.1108/00012530910973776
Selwyn N(2012) Making sense of young people, education and digital technology: the role of sociological theory Oxford Review of Education 38:81–96. https://doi.org/10.1080/03054985.2011.577949
Selwyn N, Facer K(2014) The sociology of education and digital technology: past, present and future Oxford Rev Educ 40:482–496. https://doi.org/10.1080/03054985.2014.933005
Selwyn N, Banaji S, Hadjithoma-Garstka C, Clark W(2011) Providing a platform for parents? Exploring the nature of parental engagement with school Learning Platforms J Comput Assist Learn 27:314–323. https://doi.org/10.1111/j.1365-2729.2011.00428.x
Selwyn N, Aagaard J (2020) Banning mobile phones from classrooms-an opportunity to advance understandings of technology addiction, distraction and cyberbullying. Br J Educ Technol 52. https://doi.org/10.1111/bjet.12943
Selwyn N, O’Neill C, Smith G, Andrejevic M, Gu X (2021) A necessary evil? The rise of online exam proctoring in Australian universities. Media Int Austr 1329878X2110058. https://doi.org/10.1177/1329878x211005862
Selwyn N, Pangrazio L, Nemorin S, Perrotta C (2019) What might the school of 2030 be like? An exercise in social science fiction. Learn, Media Technol 1–17. https://doi.org/10.1080/17439884.2020.1694944
Selwyn, N (2016) What works and why?* Understanding successful technology enabled learning within institutional contexts 2016 Final report Appendices (Part B). Monash University Griffith University
Sjöberg D, Holmgren R (2021) Informal workplace learning in swedish police education-a teacher perspective. Vocations and Learning. https://doi.org/10.1007/s12186-021-09267-3
Strotmann A, Zhao D (2012) Author name disambiguation: what difference does it make in author-based citation analysis? J Am Soc Inf Sci Technol 63:1820–1833
Article CAS Google Scholar
Sutherland R, Facer K, Furlong R, Furlong J(2000) A new environment for education? The computer in the home. Comput Educ 34:195–212. https://doi.org/10.1016/s0360-1315(99)00045-7
Szeto E, Cheng AY-N, Hong J-C(2015) Learning with social media: how do preservice teachers integrate YouTube and Social Media in teaching? Asia-Pac Educ Res 25:35–44. https://doi.org/10.1007/s40299-015-0230-9
Tang E, Lam C(2014) Building an effective online learning community (OLC) in blog-based teaching portfolios Int High Educ 20:79–85. https://doi.org/10.1016/j.iheduc.2012.12.002
Taskin Z, Al U(2019) Natural language processing applications in library and information science Online Inf Rev 43:676–690. https://doi.org/10.1108/oir-07-2018-0217
Tegtmeyer K, Ibsen L, Goldstein B(2001) Computer-assisted learning in critical care: from ENIAC to HAL Crit Care Med 29:N177–N182. https://doi.org/10.1097/00003246-200108001-00006
Article CAS PubMed Google Scholar
Timotheou S, Miliou O, Dimitriadis Y, Sobrino SV, Giannoutsou N, Cachia R, Moné AM, Ioannou A(2023) Impacts of digital technologies on education and factors influencing schools' digital capacity and transformation: a literature review. Educ Inf Technol 28(6):6695–6726. https://doi.org/10.1007/s10639-022-11431-8
Trujillo Maza EM, Gómez Lozano MT, Cardozo Alarcón AC, Moreno Zuluaga L, Gamba Fadul M (2016) Blended learning supported by digital technology and competency-based medical education: a case study of the social medicine course at the Universidad de los Andes, Colombia. Int J Educ Technol High Educ 13. https://doi.org/10.1186/s41239-016-0027-9
Turin O, Friesem Y(2020) Is that media literacy?: Israeli and US media scholars’ perceptions of the field J Media Lit Educ 12:132–144. https://doi.org/10.1007/s11192-009-0146-3
Van Eck NJ, Waltman L (2019) VOSviewer manual. Universiteit Leiden
Vratulis V, Clarke T, Hoban G, Erickson G(2011) Additive and disruptive pedagogies: the use of slowmation as an example of digital technology implementation Teach Teach Educ 27:1179–1188. https://doi.org/10.1016/j.tate.2011.06.004
Wang CL, Dai J, Xu LJ (2022) Big data and data mining in education: a bibliometrics study from 2010 to 2022. In 2022 7th International Conference on Cloud Computing and Big Data Analytics ( ICCCBDA ) (pp. 507-512). IEEE. https://doi.org/10.1109/icccbda55098.2022.9778874
Wang CL, Dai J, Zhu KK, Yu T, Gu XQ (2023) Understanding the continuance intention of college students toward new E-learning spaces based on an integrated model of the TAM and TTF. Int J Hum-Comput Int 1–14. https://doi.org/10.1080/10447318.2023.2291609
Wong L-H, Boticki I, Sun J, Looi C-K(2011) Improving the scaffolds of a mobile-assisted Chinese character forming game via a design-based research cycle Comput Hum Behav 27:1783–1793. https://doi.org/10.1016/j.chb.2011.03.005
Wu R, Yu Z (2023) Do AI chatbots improve students learning outcomes? Evidence from a meta-analysis. Br J Educ Technol. https://doi.org/10.1111/bjet.13334
Yang D, Zhou J, Shi D, Pan Q, Wang D, Chen X, Liu J (2022) Research status, hotspots, and evolutionary trends of global digital education via knowledge graph analysis. Sustainability 14:15157–15157. https://doi.org/10.3390/su142215157
Yu T, Dai J, Wang CL (2023) Adoption of blended learning: Chinese university students’ perspectives. Humanit Soc Sci Commun 10:390. https://doi.org/10.3390/su142215157
Yu Z (2022) Sustaining student roles, digital literacy, learning achievements, and motivation in online learning environments during the COVID-19 pandemic. Sustainability 14:4388. https://doi.org/10.3390/su14084388
Za S, Spagnoletti P, North-Samardzic A(2014) Organisational learning as an emerging process: the generative role of digital tools in informal learning practices Br J Educ Technol 45:1023–1035. https://doi.org/10.1111/bjet.12211
Zhang X, Chen Y, Hu L, Wang Y (2022) The metaverse in education: definition, framework, features, potential applications, challenges, and future research topics. Front Psychol 13:1016300. https://doi.org/10.3389/fpsyg.2022.1016300
Zhou M, Dzingirai C, Hove K, Chitata T, Mugandani R (2022) Adoption, use and enhancement of virtual learning during COVID-19. Education and Information Technologies. https://doi.org/10.1007/s10639-022-10985-x
Download references

Acknowledgements
This research was supported by the Zhejiang Provincial Social Science Planning Project, “Mechanisms and Pathways for Empowering Classroom Teaching through Learning Spaces under the Strategy of High-Quality Education Development”, the 2022 National Social Science Foundation Education Youth Project “Research on the Strategy of Creating Learning Space Value and Empowering Classroom Teaching under the background of ‘Double Reduction’” (Grant No. CCA220319) and the National College Student Innovation and Entrepreneurship Training Program of China (Grant No. 202310337023).
Author information
Authors and affiliations.
College of Educational Science and Technology, Zhejiang University of Technology, Zhejiang, China
Chengliang Wang, Xiaojiao Chen, Yidan Liu & Yuhui Jing
Graduate School of Business, Universiti Sains Malaysia, Minden, Malaysia
Department of Management, The Chinese University of Hong Kong, Hong Kong, China
College of Humanities and Social Sciences, Beihang University, Beijing, China
You can also search for this author in PubMed Google Scholar
Contributions
Conceptualization: Y.J., C.W.; methodology, C.W.; software, C.W., Y.L.; writing-original draft preparation, C.W., Y.L.; writing-review and editing, T.Y., Y.L., C.W.; supervision, X.C., T.Y.; project administration, Y.J.; funding acquisition, X.C., Y.L. All authors read and approved the final manuscript. All authors have read and approved the re-submission of the manuscript.
Corresponding author
Correspondence to Yuhui Jing .
Ethics declarations
Ethical approval.
Ethical approval was not required as the study did not involve human participants.
Informed consent
Informed consent was not required as the study did not involve human participants.
Competing interests
The authors declare no competing interests.
Additional information
Publisher’s note Springer Nature remains neutral with regard to jurisdictional claims in published maps and institutional affiliations.
Supplementary information
Rights and permissions.
Open Access This article is licensed under a Creative Commons Attribution 4.0 International License, which permits use, sharing, adaptation, distribution and reproduction in any medium or format, as long as you give appropriate credit to the original author(s) and the source, provide a link to the Creative Commons licence, and indicate if changes were made. The images or other third party material in this article are included in the article’s Creative Commons licence, unless indicated otherwise in a credit line to the material. If material is not included in the article’s Creative Commons licence and your intended use is not permitted by statutory regulation or exceeds the permitted use, you will need to obtain permission directly from the copyright holder. To view a copy of this licence, visit http://creativecommons.org/licenses/by/4.0/ .
Reprints and permissions
About this article
Cite this article.
Wang, C., Chen, X., Yu, T. et al. Education reform and change driven by digital technology: a bibliometric study from a global perspective. Humanit Soc Sci Commun 11 , 256 (2024). https://doi.org/10.1057/s41599-024-02717-y
Download citation
Received : 11 July 2023
Accepted : 17 January 2024
Published : 12 February 2024
DOI : https://doi.org/10.1057/s41599-024-02717-y
Share this article
Anyone you share the following link with will be able to read this content:
Sorry, a shareable link is not currently available for this article.
Provided by the Springer Nature SharedIt content-sharing initiative
This article is cited by
The relationship between digital literacy and job performance of university teachers: the chain mediating effect of psychological empowerment and job satisfaction.
- Jiang-hua Luo
Current Psychology (2024)
A meta-analysis of learners’ continuance intention toward online education platforms
- Chengliang Wang
Education and Information Technologies (2024)
Quick links
- Explore articles by subject
- Guide to authors
- Editorial policies

Advertisement
Artificial Intelligence in Education (AIEd): a high-level academic and industry note 2021
- Original Research
- Open access
- Published: 07 July 2021
- Volume 2 , pages 157–165, ( 2022 )
Cite this article
You have full access to this open access article
- Muhammad Ali Chaudhry ORCID: orcid.org/0000-0003-0154-2613 1 &
- Emre Kazim 2
60k Accesses
102 Citations
25 Altmetric
Explore all metrics
In the past few decades, technology has completely transformed the world around us. Indeed, experts believe that the next big digital transformation in how we live, communicate, work, trade and learn will be driven by Artificial Intelligence (AI) [ 83 ]. This paper presents a high-level industrial and academic overview of AI in Education (AIEd). It presents the focus of latest research in AIEd on reducing teachers’ workload, contextualized learning for students, revolutionizing assessments and developments in intelligent tutoring systems. It also discusses the ethical dimension of AIEd and the potential impact of the Covid-19 pandemic on the future of AIEd’s research and practice. The intended readership of this article is policy makers and institutional leaders who are looking for an introductory state of play in AIEd.
Similar content being viewed by others
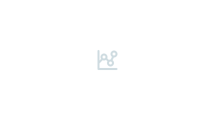
The Birth of IJAIED
Exploring the Impact of Artificial Intelligence in Education: A Comprehensive Review and Future Directions
Evolution and revolution in artificial intelligence in education, explore related subjects.
- Artificial Intelligence
Avoid common mistakes on your manuscript.
1 Introduction
Artificial Intelligence (AI) is changing the world around us [ 42 ]. As a term it is difficult to define even for experts because of its interdisciplinary nature and evolving capabilities. In the context of this paper, we define AI as a computer system that can achieve a particular task through certain capabilities (like speech or vision) and intelligent behaviour that was once considered unique to humans [ 54 ]. In more lay terms we use the term AI to refer to intelligent systems that can automate tasks traditionally carried out by humans. Indeed, we read AI within the continuation of the digital age, with increased digital transformation changing the ways in which we live in the world. With such change the skills and knowhow of people must reflect the new reality and within this context, the World Economic Forum identified sixteen skills, referred to as twenty-first century skills necessary for the future workforce [ 79 ]. This includes skills such as technology literacy, communication, leadership, curiosity, adaptability, etc. These skills have always been important for a successful career, however, with the accelerated digital transformation of the past 2 years and the focus on continuous learning in most professional careers, these skills are becoming necessary for learners.
AI will play a very important role in how we teach and learn these new skills. In one dimension, ‘AIEd’ has the potential to dramatically automate and help track the learner’s progress in all these skills and identify where best a human teacher’s assistance is needed. For teachers, AIEd can potentially be used to help identify the most effective teaching methods based on students’ contexts and learning background. It can automate monotonous operational tasks, generate assessments and automate grading and feedback. AI does not only impact what students learn through recommendations, but also how they learn, what are the learning gaps, which pedagogies are more effective and how to retain learner’s attention. In these cases, teachers are the ‘human-in-the-loop’, where in such contexts, the role of AI is only to enable more informed decision making by teachers, by providing them predictions about students' performance or recommending relevant content to students after teachers' approval. Here, the final decision makers are teachers.
Segal et al. [ 58 ] developed a system named SAGLET that utilized ‘human-in-the-loop’ approach to visualize and model students’ activities to teachers in real-time enabling them to intervene more effectively as and when needed. Here the role of AI is to empower the teachers enabling them to enhance students’ learning outcomes. Similarly, Rodriguez et al. [ 52 ] have shown how teachers as ‘human-in-the-loop’ can customize multimodal learning analytics and make them more effective in blended learning environments.
Critically, all these achievements are completely dependent on the quality of available learner data which has been a long-lasting challenge for ed-tech companies, at least until the pandemic. Use of technology in educational institutions around the globe is increasing [ 77 ], however, educational technology (ed-tech) companies building AI powered products have always complained about the lack of relevant data for training algorithms. The advent and spread of Covid in 2019 around the world pushed educational institutions online and left them at the mercy of ed-tech products to organize content, manage operations, and communicate with students. This shift has started generating huge amounts of data for ed-tech companies on which they can build AI systems. According to a joint report: ‘Shock to the System’, published by Educate Ventures and Cambridge University, optimism of ed-tech companies about their own future increased during the pandemic and their most pressing concern became recruitment of too many customers to serve effectively [ 15 ].
Additionally, most of the products and solutions provided by ed-tech start-ups lack the quality and resilience to cope with intensive use of several thousands of users. Product maturity is not ready for huge and intense demand as discussed in Sect. “ Latest research ” below. We also discuss some of these products in detail in Sect. “ Industry’s focus ” below. How do we mitigate the risks of these AI powered products and who monitors the risk? (we return to this theme in our discussion of ethics—Sect. “ Ethical AIEd ”).
This paper is a non-exhaustive overview of AI in Education that presents a brief survey of the latest developments of AI in Education. It begins by discussing different aspects of education and learning where AI is being utilized, then turns to where we see the industry’s current focus and then closes with a note on ethical concerns regarding AI in Education. This paper also briefly evaluates the potential impact of the pandemic on AI’s application in education. The intended readership of this article is the policy community and institutional executives seeking an instructive introduction to the state of play in AIEd. The paper can also be read as a rapid introduction to the state of play of the field.
2 Latest research
Most work within AIEd can be divided into four main subdomains. In this section, we survey some of the latest work in each of these domains as case studies:
Reducing teachers’ workload: the purpose of AI in Education is to reduce teachers’ workload without impacting learning outcomes
Contextualized learning for students: as every learner has unique learning needs, the purpose of AI in Education is to provide customized and/or personalised learning experiences to students based on their contexts and learning backgrounds.
Revolutionizing assessments: the purpose of AI in Education is to enhance our understanding of learners. This not only includes what they know, but also how they learn and which pedagogies work for them.
Intelligent tutoring systems (ITS): the purpose of AI in Education is to provide intelligent learning environments that can interact with students, provide customized feedback and enhance their understanding of certain topics
2.1 Reducing teachers’ workload
Recent research in AIEd is focusing more on teachers than other stakeholders of educational institutions, and this is for the right reasons. Teachers are at the epicenter of every learning environment, face to face or virtual. Participatory design methodologies ensure that teachers are an integral part of the design of new AIEd tools, along with parents and learners [ 45 ]. Reducing teachers’ workload has been a long-lasting challenge for educationists, hoping to achieve more affective teaching in classrooms by empowering the teachers and having them focus more on teaching than the surrounding activities.
With the focus on online education during the pandemic and emergence of new tools to facilitate online learning, there is a growing need for teachers to adapt to these changes. Importantly, teachers themselves are having to re-skill and up-skill to adapt to this age, i.e. the new skills that teachers need to develop to fully utilize the benefits of AIEd [ 39 ]. First, they need to become tech savvy to understand, evaluate and adapt new ed-tech tools as they become available. They may not necessarily use these tools, but it is important to have an understanding of what these tools offer and if they share teachers’ workload. For example, Zoom video calling has been widely used during the pandemic to deliver lessons remotely. Teachers need to know not only how to schedule lessons on Zoom, but also how to utilize functionalities like breakout rooms to conduct group work and Whiteboard for free style writing. Second, teachers will also need to develop analytical skills to interpret the data that are visualized by these ed-tech tools and to identify what kind of data and analytics tools they need to develop a better understanding of learners. This will enable teachers to get what they exactly need from ed-tech companies and ease their workload. Third, teachers will also need to develop new team working, group and management skills to accommodate new tools in their daily routines. They will be responsible for managing these new resources most efficiently.
Selwood and Pilkington [ 61 ] showed that the use of Information and Communication Technologies (ICT) leads to a reduction in teachers’ workload if they use it frequently, receive proper training on how to use ICT and have access to ICT in home and school. During the pandemic, teachers have been left with no options other than online teaching. Spoel et al. [ 76 ] have shown that the previous experience with ICT did not play a significant role in how they dealt with the online transition during pandemic. Suggesting that the new technologies are not a burden for teachers. It is early to draw any conclusions on the long-term effects of the pandemic on education, online learning and teachers’ workload. Use of ICT during the pandemic may not necessarily reduce teacher workload, but change its dynamics.
2.2 Contextualized learning for students
Every learner has unique learning contexts based on their prior knowledge about the topic, social background, economic well-being and emotional state [ 41 ]. Teaching is most effective when tailored to these changing contexts. AIEd can help in identifying the learning gaps in each learner, offer content recommendations based on that and provide step by step solutions to complex problems. For example, iTalk2Learn is an opensource platform that was developed by researchers to support math learning among students between 5 and 11 years of age [ 22 ]. This tutor interacted with students through speech, identified when students were struggling with fractions and intervened accordingly. Similarly, Pearson has launched a calculus learning tool called AIDA that provides step by step guidance to students and helps them complete calculus tasks. Use of such tools by young students also raises interesting questions about the illusion of empathy that learners may develop towards such educational bots [ 73 ].
Open Learner Models [ 12 , 18 ] have been widely used in AIEd to facilitate learners, teachers and parents in understanding what learners know, how they learn and how AI is being used to enhance learning. Another important construct in understanding learners is self-regulated learning [ 10 , 68 ]. Zimmerman and Schunk [ 85 ] define self-regulated learning as learner’s thoughts, feelings and actions towards achieving a certain goal. Better understanding of learners through open learner models and self-regulated learning is the first step towards contextualized learning in AIEd. Currently, we do not have completely autonomous digital tutors like Amazon’s Alexa or Apple’s Siri for education but domain specific Intelligent Tutoring Systems (ITS) are also very helpful in identifying how much students know, where they need help and what type of pedagogies would work for them.
There are a number of ed-tech tools available to develop basic literacy skills in learners like double digit division or improving English grammar. In future, AIEd powered tools will move beyond basic literacy to develop twenty-first century skills like curiosity [ 49 ], initiative and creativity [ 51 ], collaboration and adaptability [ 36 ].
2.3 Revolutionizing assessments
Assessment in educational context refers to ‘any appraisal (or judgement or evaluation) of a student’s work or performance’ [ 56 ]. Hill and Barber [ 27 ] have identified assessments as one of the three pillars of schooling along with curriculum and learning and teaching. The purpose of modern assessments is to evaluate what students know, understand and can do. Ideally, assessments should take account of the full range of student abilities and provide useful information about learning outcomes. However, every learner is unique and so are their learning paths. How can standardized assessment be used to evaluate every student, with distinct capabilities, passions and expertise is a question that can be posed to broader notions of educational assessment. According to Luckin [ 37 ] from University College London, ‘AI would provide a fairer, richer assessment system that would evaluate students across a longer period of time and from an evidence-based, value-added perspective’.
AIAssess is an example of an intelligent assessment tool that was developed by researchers at UCL Knowledge lab [ 38 , 43 ]. It assessed students learning math and science based on three models: knowledge model, analytics model and student model. Knowledge component stored the knowledge about each topic, the analytics component analyzed students’ interactions and the student model tracked students’ progress on a particular topic. Similarly, Samarakou et al. [ 57 ] have developed an AI assessment tool that also does qualitative evaluation of students to reduce the workload of instructors who would otherwise spend hours evaluating every exercise. Such tools can be further empowered by machine learning techniques such as semantic analysis, voice recognition, natural language processing and reinforcement learning to improve the quality of assessments.
2.4 Intelligent tutoring systems (ITS)
An intelligent tutoring system is a computer program that tries to mimic a human teacher to provide personalized learning to students [ 46 , 55 ]. The concept of ITS in AIEd is decades old [ 9 ]. There have always been huge expectations from ITS capabilities to support learning. Over the years, we have observed that there has been a significant contrast between what ITS were envisioned to deliver and what they have actually been capable of doing [ 4 ].
A unique combination of domain models [ 78 ], pedagogical models [ 44 ] and learner models [ 20 ] were expected to provide contextualized learning experiences to students with customized content, like expert human teachers [ 26 , 59 , 65 ],. Later, more models were introduced to enhance students' learning experience like strategy model, knowledge-base model and communication model [ 7 ]. It was expected that an intelligent tutoring system would not just teach, but also ensure that students have learned. It would care for students [ 17 ]. Similar to human teachers, ITS would improve with time. They would learn from their experiences, ‘understand’ what works in which contexts and then help students accordingly [ 8 , 60 ].
In recent years, ITS have mostly been subject and topic specific like ASSISTments [ 25 ], iTalk2Learn [ 23 ] and Aida Calculus. Despite being limited in terms of the domain that a particular intelligent tutoring system addresses, they have proven to be effective in providing relevant content to students, interacting with students [ 6 ] and improving students’ academic performance [ 18 , 41 ]. It is not necessary that ITS would work in every context and facilitate every teacher [ 7 , 13 , 46 , 48 ]. Utterberg et al. [78] showed why teachers have abandoned technology in some instances because it was counterproductive. They conducted a formative intervention with sixteen secondary school mathematics teachers and found systemic contradictions between teachers’ opinions and ITS recommendations, eventually leading to the abandonment of the tool. This highlights the importance of giving teachers the right to refuse AI powered ed-tech if they are not comfortable with it.
Considering a direct correlation between emotions and learning [ 40 ] recently, ITS have also started focusing on emotional state of students while learning to offer a more contextualized learning experience [ 24 ].
2.5 Popular conferences
To reflect on the increasing interest and activity in the space of AIEd, some of the most popular conferences in AIEd are shown in Table 1 below. Due to the pandemic all these conferences will be available virtually in 2021 as well. The first international workshop on multimodal artificial intelligence in education is being organized at AIEd [74] conference to promote the importance of multimodal data in AIEd.
3 Industry’s focus
In this section, we introduce the industry focus in the area of AIEd by case-studying three levels of companies start-up level, established/large company and mega-players (Amazon, Cisco). These companies represent different levels of the ecosystem (in terms of size).
3.1 Start-ups
There have been a number of ed-tech companies that are leading the AIEd revolution. New funds are also emerging to invest in ed-tech companies and to help ed-tech start-ups in scaling their products. There has been an increase in investor interest [ 21 ]. In 2020 the amount of investment raised by ed-tech companies more than doubled compared to 2019 (according to Techcrunch). This shows another dimension of pandemic’s effect on ed-tech. With an increase in data coming in during the pandemic, it is expected that industry’s focus on AI powered products will increase.
EDUCATE, a leading accelerator focused on ed-tech companies supported by UCL Institute of Education and European Regional Development Fund was formed to bring research and evidence at the centre of product development for ed-tech. This accelerator has supported more than 250 ed-tech companies and 400 entrepreneurs and helped them focus on evidence-informed product development for education.
Number of ed-tech companies are emerging in this space with interesting business models. Third Space Learning offers maths intervention programs for primary and secondary school students. The company aims to provide low-cost quality tuition to support pupils from disadvantaged backgrounds in UK state schools. They have already offered 8,00,000 h of teaching to around 70,000 students, 50% of who were eligible for free meals. Number of mobile apps like Kaizen Languages, Duolingo and Babbel have emerged that help individuals in learning other languages.
3.2 Established players
Pearson is one of the leading educational companies in the world with operations in more than 70 countries and more than 22,000 employees worldwide. They have been making a transition to digital learning and currently generate 66% of their annual revenue from it. According to Pearson, they have built world’s first AI powered calculus tutor called Aida which is publicly available on the App Store. But, its effectiveness in improving students’ calculus skills without any human intervention is still to be seen.
India based ed-tech company known for creating engaging educational content for students raised investment at a ten billion dollar valuation last year [ 70 ]. Century tech is another ed-tech company that is empowering learning through AI. They claim to use neuroscience, learning science and AI to personalize learning and identifying the unique learning pathways for students in 25 countries. They make more than sixty thousand AI powered smart recommendations to learners every day.
Companies like Pearson and Century Tech are building great technology that is impacting learners across the globe. But the usefulness of their acclaimed AI in helping learners from diverse backgrounds, with unique learning needs and completely different contexts is to be proven. As discussed above, teachers play a very important role on how their AI is used by learners. For this, teacher training is vital to fully understand the strengths and weaknesses of these products. It is very important to have an awareness of where these AI products cannot help or can go wrong so teachers and learners know when to avoid relying on them.
In the past few years, the popularity of Massive Online Open Courses (MOOCS) has grown exponentially with the emergence of platforms like Coursera, Udemy, Udacity, LinkedIn Learning and edX [ 5 , 16 , 28 ]. AI can be utilized to develop a better understanding of learner behaviour on MOOCS, produce better content and enhance learning outcomes at scale. Considering these platforms are collecting huge amounts of data, it will be interesting to see the future applications of AI in offering personalized learning and life-long learning solutions to their users [ 81 ].
3.3 Mega-players
Seeing the business potential of AIEd and the kind of impact it can have on the future of humanity, some of the biggest tech companies around the globe are moving into this space. The shift to online education during the pandemic boosted the demand for cloud services. Amazon’s AWS (Amazon Web Services) as a leader in cloud services provider facilitated institutions like Instituto Colombiano para la Evaluacion de la Educacion (ICFES) to scale their online examination service for 70,000 students. Similarly, LSE utilized AWS to scale their online assessments for 2000 students [ 1 , 3 ].
Google’s CEO Sunder Pichai stated that the pandemic offered an incredible opportunity to re-imagine education. Google has launched more than 50 new software tools during the pandemic to facilitate remote learning. Google Classroom which is a part of Google Apps for Education (GAFE) is being widely used by schools around the globe to deliver education. Research shows that it improves class dynamics and helps with learner participation [ 2 , 29 , 62 , 63 , 69 ].
Before moving onto the ethical dimensions of AIEd, it is important to conclude this section by noting an area that is of critical importance to processing industry and services. Aside from these three levels of operation (start-up, medium, and mega companies), there is the question of development of the AIEd infrastructure. As Luckin [41] points out, “True progress will require the development of an AIEd infrastructure. This will not, however, be a single monolithic AIEd system. Instead, it will resemble the marketplace that has been developed for smartphone apps: hundreds and then thousands of individual AIEd components, developed in collaboration with educators, conformed to uniform international data standards, and shared with researchers and developers worldwide. These standards will enable system-level data collation and analysis that help us learn much more about learning itself and how to improve it”.
4 Ethical AIEd
With a number of mishaps in the real world [ 31 , 80 ], ethics in AI has become a real concern for AI researchers and practitioners alike. Within computer science, there is a growing overlap with the broader Digital Ethics [ 19 ] and the ethics and engineering focused on developing Trustworthy AI [ 11 ]. There is a focus on fairness, accountability, transparency and explainability [ 33 , 82 , 83 , 84 ]. Ethics in AI needs to be embedded in the entire development pipeline, from the decision to start collecting data till the point when the machine learning model is deployed in production. From an engineering perspective, Koshiyama et al. [ 35 ] have identified four verticals of algorithmic auditing. These include performance and robustness, bias and discrimination, interpretability and explainability and algorithmic privacy.
In education, ethical AI is crucial to ensure the wellbeing of learners, teachers and other stakeholders involved. There is a lot of work going on in AIEd and AI powered ed-tech tools. With the influx of large amounts of data due to online learning during the pandemic, we will most likely see an increasing number of AI powered ed-tech products. But ethics in AIEd is not a priority for most ed-tech companies and schools. One of the reasons for this is the lack of awareness of relevant stakeholders regarding where AI can go wrong in the context of education. This means that the drawbacks of using AI like discrimination against certain groups due to data deficiencies, stigmatization due to reliance on certain machine learning modelling deficiencies and exploitation of personal data due to lack of awareness can go unnoticed without any accountability.
An AI wrongly predicting that a particular student will not perform very well in end of year exams or might drop out next year can play a very important role in determining that student’s reputation in front of teachers and parents. This reputation will determine how these teachers and parents treat that learner, resulting in a huge psychological impact on that learner, based on this wrong description by an AI tool. One high-profile case of harm was in the use of an algorithm to predict university entry results for students unable to take exams due to the pandemic. The system was shown to be biased against students from poorer backgrounds. Like other sectors where AI is making a huge impact, in AIEd this raises an important ethical question regarding giving students the freedom to opt out of AI powered predictions and automated evaluations.
The ethical implications of AI in education are dependent on the kind of disruption AI is doing in the ed-tech sector. On the one hand, this can be at an individual level for example by recommending wrong learning materials to students, or it can collectively impact relationships between different stakeholders such as how teachers perceive learners’ progress. This can also lead to automation bias and issues of accountability [ 67 ] where teachers begin to blindly rely on AI tools and prefer the tool’s outcomes over their own better judgement, whenever there is a conflict.
Initiatives have been observed in this space. For example, Professor Rose Luckin, professor of learner centered design at University College London along with Sir Anthony Seldon, vice chancellor of the University of Buckingham and Priya Lakhani, founder and CEO of Century Tech founded the Institute of Ethical AI in Education (IEAIEd) [ 72 ] to create awareness and promote the ethical aspects of AI in education. In its interim report, the institute identified seven different requirements for ethical AI to mitigate any kind of risks for learners. This included human agency and oversight to double-check AI’s performance, technical robustness and safety to prevent AI going wrong with new data or being hacked; diversity to ensure similar distribution of different demographics in data and avoid bias; non-discrimination and fairness to prevent anyone from being unfairly treated by AI; privacy and data governance to ensure everyone has the right to control their data; transparency to enhance the understanding of AI products; societal and environmental well-being to ensure that AI is not causing any harm and accountability to ensure that someone takes the responsibility for any wrongdoings of AI. Recently, the institute has also published a framework [ 71 ] for educators, schools and ed-tech companies to help them with the selection of ed-tech products with various ethical considerations in mind, like ethical design, transparency, privacy etc.
With the focus on online learning during the pandemic, and more utilization of AI powered ed-tech tools, risks of AI going wrong have increased significantly for all the stakeholders including ed-tech companies, schools, teachers and learners. A lot more work needs to be done on ethical AI in learning contexts to mitigate these risks, including assessment balancing risks and opportunities.
UNESCO published ‘Beijing Consensus’ on AI and Education that recommended member states to take a number of actions for the smooth and positively impactful integration of AI with education [ 74 ]. International bodies like EU have also recently published a set of draft guidelines under the heading of EU AI Act to ban certain uses of AI and categorize some as ‘high risk’ [ 47 ].
5 Future work
With the focus on online education due to Covid’19 in the past year, it will be consequential to see what AI has to offer for education with vast amounts of data being collected online through Learning Management Systems (LMS) and Massive Online Open Courses (MOOCS).
With this influx of educational data, AI techniques such as reinforcement learning can also be utilized to empower ed-tech. Such algorithms perform best with the large amounts of data that was limited to very few ed-tech companies in 2021. These algorithms have achieved breakthrough performance in multiple domains including games [ 66 ], healthcare [ 14 ] and robotics [ 34 ]. This presents a great opportunity for AI’s applications in education for further enhancing students’ learning outcomes, reducing teachers’ workloads [ 30 ] and making learning personalized [ 64 ], interactive and fun [ 50 , 53 ] for teachers and students.
With a growing number of AI powered ed-tech products in future, there will also be a lot of research on ethical AIEd. The risks of AI going wrong in education and the psychological impact this can have on learners and teachers is huge. Hence, more work needs to be done to ensure robust and safe AI products for all the stakeholders.
This can begin from the ed-tech companies sharing detailed guidelines for using AI powered ed-tech products, particularly specifying when not to rely on them. This includes the detailed documentation of the entire machine learning development pipeline with the assumptions made, data processing approaches used and the processes followed for selecting machine learning models. Regulators can play a very important role in ensuring that certain ethical principles are followed in developing these AI products or there are certain minimum performance thresholds that these products achieve [ 32 ].
6 Conclusion
AIEd promised a lot in its infancy around 3 decades back. However, there are still a number of AI breakthroughs required to see that kind of disruption in education at scale (including basic infrastructure). In the end, the goal of AIEd is not to promote AI, but to support education. In essence, there is only one way to evaluate the impact of AI in Education: through learning outcomes. AIEd for reducing teachers’ workload is a lot more impactful if the reduced workload enables teachers to focus on students’ learning, leading to better learning outcomes.
Cutting edge AI by researchers and companies around the world is not of much use if it is not helping the primary grade student in learning. This problem becomes extremely challenging because every learner is unique with different learning pathways. With the recent developments in AI, particularly reinforcement learning techniques, the future holds exciting possibilities of where AI will take education. For impactful AI in education, learners and teachers always need to be at the epicenter of AI development.
About Amazon.: Helping 7,00,000 students transition to remote learning. https://www.aboutamazon.com/news/community/helping-700-000-students-transition-to-remote-learning (2020)
Al-Maroof, R.A.S., Al-Emran, M.: Students acceptance of google classroom: an exploratory study using PLS–SEM approach. Int. J. Emerg. Technol Learn. (2018). https://doi.org/10.3991/ijet.v13i06.8275
Article Google Scholar
Amazon Web Services, Inc. (n.d.).: Amazon Web Services, Inc. https://pages.awscloud.com/whitepaper-emerging-trends-in-education.html (2020)
Baker, R.S.: Stupid tutoring systems, intelligent humans. Int. J. Artif. Intell. Educ. 26 (2), 600–614 (2016)
Baturay, M.H.: An overview of the world of MOOCs. Procedia. Soc. Behav. Sci. 174 , 427–433 (2015)
Baylari, A., Montazer, G.A.: Design a personalized e-learning system based on item response theory and artificial neural network approach. Expert. Syst. Appl. 36 (4), 8013–8021 (2009)
Beck, J., Stern, M., Haugsjaa, E.: Applications of AI in education. Crossroads 3 (1), 11–15 (1996). https://doi.org/10.1016/j.eswa.2008.10.080
Beck, J.E.: Modeling the Student with Reinforcement Learning. Proceedings of the Machine learning for User Modeling Workshop at the Sixth International Conference on User Modeling (1997)
Beck, J.E., Woolf, B.P., Beal, C.R.: ADVISOR: A machine learning architecture for intelligent tutor construction. Proceedings of the 7th National Conference on Artificial Intelligence, New York, ACM, 552–557 (2000)
Boekaerts, M.: Self-regulated learning: where we are today. Int. J. Educ. Res. 31 (6), 445–457 (1999)
Brundage, M., Avin, S., Wang, J., Belfield, H., Krueger, G., Hadfield, G., Maharaj, T.: Toward trustworthy AI development: mechanisms for supporting verifiable claims. arXiv preprint arXiv:2004.07213 (2020)
Bull, S., Kay, J.: Open learner models. In: Nkambou, R., Bourdeau, J., Mizoguchi, R. (eds.) Studies in computational intelligence, pp. 301–322. Springer, Berlin (2010)
Google Scholar
Cunha-Perez, C., Arevalillo-Herraez, M., Marco-Gimenez, L., Arnau, D.: On incorporating affective support to an intelligent tutoring system: an empirical study. IEEE. R. Iberoamericana. De. Tecnologias. Del. Aprendizaje. 13 (2), 63–69 (2018)
Callaway, E.: “It will change everything”: DeepMind’s AI makes gigantic leap in solving protein structures. Nature. https://www.nature.com/articles/d41586-020-03348-4 . (2020)
Cambridge University Press and Educate Ventures. Shock to the system: lessons from Covid-19 Volume 1: Implications and recommendations. https://www.cambridge.org/pk/files/1616/1349/4545/Shock_to_the_System_Lessons_from_Covid19_Volume_1.pdf (2021). Accessed 12 Apr 2021
Deng, R., Benckendorff, P., Gannaway, D.: Progress and new directions for teaching and learning in MOOCs. Comput. Educ. 129 , 48–60 (2019)
Erümit, A.K., Çetin, İ: Design framework of adaptive intelligent tutoring systems. Educ. Inf. Technol. 25 (5), 4477–4500 (2020)
Fang, Y., Ren, Z., Hu, X., Graesser, A.C.: A meta-analysis of the effectiveness of ALEKS on learning. Educ. Psychol. 39 (10), 1278–1292 (2019)
Floridi, L.: Soft ethics, the governance of the digital and the general data protection regulation. Philos. Trans. R. Soc. A. Math. Phys. Eng. Sci. 376 (2133), 20180081 (2018)
Goldstein, I.J.: The genetic graph: a representation for the evolution of procedural knowledge. Int. J. Man. Mach. Stud. 11 (1), 51–77 (1979)
Goryachikh, S.P., Sozinova, A.A., Grishina, E.N., Nagovitsyna, E.V.: Optimisation of the mechanisms of managing venture investments in the sphere of digital education on the basis of new information and communication technologies: audit and reorganisation. IJEPEE. 13 (6), 587–594 (2020)
Grawemeyer, B., Gutierrez-Santos, S., Holmes, W., Mavrikis, M., Rummel, N., Mazziotti, C., Janning, R.: Talk, tutor, explore, learn: intelligent tutoring and exploration for robust learning, p. 2015. AIED, Madrid (2015)
Hansen, A., Mavrikis, M.: Learning mathematics from multiple representations: two design principles. ICTMT-12, Faro (2015)
Hasan, M.A., Noor, N.F.M., Rahman, S.S.A., Rahman, M.M.: The transition from intelligent to affective tutoring system: a review and open issues. IEEE Access (2020). https://doi.org/10.1109/ACCESS.2020.3036990
Heffernan, N.T., Heffernan, C.L.: The ASSISTments ecosystem: building a platform that brings scientists and teachers together for minimally invasive research on human learning and teaching. Int. J. Artif. Intell. Educ. (2014). https://doi.org/10.1007/s40593-014-0024-x
Heffernan, N.T., Koedinger, K.R.: An intelligent tutoring system incorporating a model of an experienced human tutor. Proceedings of the 6th International Conference on Intelligent Tutoring Systems, 2363, p 596–608, (2002)
Hill, P., Barber, M.: Preparing for a Renaissance in Assessment. Pearson, London (2014)
Hollands, F.M., Tirthali, D.: Why do institutions offer MOOCs? Online Learning 18 (3), 3 (2014)
Iftakhar, S.: Google classroom: what works and how. J. Educ. Soc. Sci. 3 (1), 12–18 (2016)
Iglesias, A., Martínez, P., Aler, R., Fernández, F.: Reinforcement learning of pedagogical policies in adaptive and intelligent educational systems. Knowl. Based. Syst. 22 (4), 266–270 (2009)
Johnson, D.G., Verdicchio, M.: AI, agency and responsibility: the VW fraud case and beyond. Ai. Soc. 34 (3), 639–647 (2019)
Kazim, E., Denny, D.M.T., Koshiyama, A.: AI auditing and impact assessment: according to the UK information commissioner’s office. AI. Ethics. 1 , 1–10 (2021)
Kazim, E., Koshiyama, A.: A High-Level Overview of AI Ethics. SSRN J (2020). https://doi.org/10.2139/ssrn.3609292
Kober, J., Bagnell, J.A., Peters, J.: Reinforcement learning in robotics: a survey. Int. J. Robot. Res. 32 (11), 1238–1274 (2013)
Koshiyama, A., Kazim, E., Treleaven, P., Rai, P., Szpruch, L., Pavey, G., Ahamat, G., Leutner, F., Goebel, R., Knight, A., Adams, J., Hitrova, C., Barnett, J., Nachev, P., Barber, D., Chamorro-Premuzic, T., Klemmer, K., Gregorovic, M., Khan, S., Lomas, E.: Towards algorithm auditing a survey on managing legal ethical and technological risks of AI, ML and associated algorithms. SSRN J (2021). https://doi.org/10.2139/ssrn.3778998
LaPierre, J.: How AI Enhances Collaborative Learning. Filament Games (2018). https://www.filamentgames.com/blog/how-ai-enhances-collaborative-learning/ . Accessed 12 Apr 2021
Luckin, R.: Towards artificial intelligence-based assessment systems. Nat. Hum. Behav. (2017). https://doi.org/10.1038/s41562-016-0028
Luckin, R., du Boulay, B.: Int. J. Artif. Intell. Educ. 26 , 416–430 (2016)
Luckin, R., Holmes, W., Griffiths, M., Pearson, L.: Intelligence Unleashed An argument for AI in Education. https://static.googleusercontent.com/media/edu.google.com/en//pdfs/Intelligence-Unleashed-Publication.pdf (2016)
Barron-Estrada M.L., Zatarain-Cabada, R., Oramas-Bustillos, R., Gonzalez-Hernandez, F.: Sentiment analysis in an affective intelligent tutoring system. Proc. IEEE 17th Int. Conf. Adv. Learn. Technol. (ICALT), Timisoara pp. 394–397 2017.
Ma, W., Adesope, O., Nesbit, J.C., Liu, Q.: Intelligent tutoring systems and learning outcomes: a meta-analysis. J. Educ. Psychol. 106 (4), 901–918 (2014)
Makridakis, S.: The forthcoming Artificial Intelligence (AI) revolution: Its impact on society and firms. Futures 90 , 46–60 (2017)
Mavrikis, M.: Int. J. Artif. Intell. Tools. 19 , 733–753 (2010)
Merrill, D.C., Reiser, B.J., Ranney, M., Trafton, J.G.: Effective tutoring techniques: a comparison of human tutors and intelligent tutoring systems. J. Learn. Sci. 2 (3), 277–305 (1992)
Moeini, A.: Theorising Evidence-Informed Learning Technology Enterprises: A Participatory Design-Based Research Approach. Doctoral dissertation, UCL University College London, London, (2020)
Mohamed, H., Lamia, M.: Implementing flipped classroom that used an intelligent tutoring system into learning process. Comput. Educ. 124 , 62–76 (2018). https://doi.org/10.1016/j.compedu.2018.05.011
Mueller, B.: The Artificial Intelligence Act: A Quick Explainer. [online] Center for Data Innovation (2021). https://datainnovation.org/2021/05/the-artificial-intelligence-act-a-quick-explainer/ . Accessed 12 Apr 2021
Murray, M.C., Pérez, J.: Informing and performing: A study comparing adaptive learning to traditional learning. Inform. Sci. J. 18 , 111–125 (2015)
Oudeyer, P-Y.: Computational Theories of Curiosity-Driven Learning. https://arxiv.org/pdf/1802.10546.pdf (2018)
Park, H.W., Grover, I., Spaulding, S., Gomez, L., Breazeal, C.: A model-free affective reinforcement learning approach to personalization of an autonomous social robot companion for early literacy education. AAAI. 33 (1), 687–694 (2019)
Resnick, M., Robinson, K.: Lifelong kindergarten: cultivating creativity through projects, passion, peers, and play. MIT press, Cambridge (2017)
Book Google Scholar
Rodríguez-Triana, M.J., Prieto, L.P., Martínez-Monés, A., Asensio-Pérez, J.I. and Dimitriadis, Y.: The teacher in the loop: Customizing multimodal learning analytics for blended learning. In Proceedings of the 8th international conference on learning analytics and knowledge. pp 417–426 (2018)
Rowe, J.P., Lester, J.C.: Improving student problem solving in narrative-centered learning environments: a modular reinforcement learning framework. In International Conference on Artificial Intelligence in Education. pp. 419–428. Springer, Cham (2015)
Russell, S.J., Norvig, P., Davis, E.: Artificial intelligence: a modern approach. Prentice Hall, Upper Saddle River (2010)
MATH Google Scholar
Jiménez, S., Juárez-Ramírez, R., Castillo, V.H., Licea, G., Ramírez-Noriega, A., Inzunza, S.: A feedback system to provide affective support to students. Comput. Appl. Eng. Educ. 26 (3), 473–483 (2018)
Sadler, D.R.: Formative assessment in the design of instructional systems. Instr. Sci. 18 , 119–144 (1989)
Samarakou, M., Fylladitakis, E., Prentakis, P., Athineos, S.: Implementation of artificial intelligence assessment in engineering laboratory education. https://files.eric.ed.gov/fulltext/ED557263.pdf (2014). Accessed 24 Feb 2021
Segal, A., Hindi, S., Prusak, N., Swidan, O., Livni, A., Palatnic, A., Schwarz, B.: Keeping the teacher in the loop: Technologies for monitoring group learning in real-time. In International Conference on Artificial Intelligence in Education. pp. 64–76. Springer, Cham (2017)
Self, J. A. (1990). Theoretical foundations of intelligent tutoring systems. J. Artif. Intell
Self, J.A.: The defining characteristics of intelligent tutoring systems research: ITSs care, precisely. IJAIEd. 10 , 350–364 (1998)
Selwood, I., Pilkington, R.: Teacher workload: using ICT to release time to teach. Educ. Rev. 57 (2), 163–174 (2005)
Shaharanee, I.N.M., Jamil, J.M. and Rodzi, S.S.M.:æ Google classroom as a tool for active learning. AIP Conference Proceedings, 1761 (1), pp. 020069, AIP Publishing LLC, College Park (2016)
Shaharanee, I.N.M., Jamil, J.M., Rodzi, S.S.M.: The application of Google Classroom as a tool for teaching and learning. J. Telecommun. Electron. Comp. Eng. 8 (10), 5–8 (2016)
Shawky, D., Badawi, A.: Towards a personalized learning experience using reinforcement learning. In: Hassanien, A.E. (ed.) Machine learning paradigms Theory and application, pp. 169–187. Springer (2019)
Shute, V.J. (1991). Rose garden promises of intelligent tutoring systems: blossom or thorn. NASA, Lyndon B. Johnson Space Center, Fourth Annual Workshop on Space Operations Applications and Research (SOAR 90) . Available at: https://ntrs.nasa.gov/citations/19910011382 . Accessed 4 July 2021
Silver, D., Huang, A., Maddison, C.J., Guez, A., Sifre, L., Van Den Driessche, G., Schrittwieser, J., Antonoglou, I., Panneershelvam, V., Lanctot, M., Dieleman, S.: Mastering the game of Go with deep neural networks and tree search. Nature 529 (7587), 484–489 (2016)
Skitka, L.J., Mosier, K., Burdick, M.D.: Accountability and automation bias. Int. J. Hum. Comput. Stud. 52 (4), 701–717 (2000)
Steenbergen-Hu, S., Cooper, H.: A meta-analysis of the effectiveness of intelligent tutoring systems on K–12 students’ mathematical learning. J. Educ. Psychol. 105 (4), 970–987 (2013)
Sudarsana, I.K., Putra, I.B., Astawa, I.N.T., Yogantara, I.W.L.: The use of google classroom in the learning process. J. Phys. Conf. Ser 1175 (1), 012165 (2019)
TechCrunch. Indian education startup Byju’s is fundraising at a $10B valuation. https://techcrunch.com/2020/05/01/indian-education-startup-byjus-is-fundraising-at-a-10b-valuation/ (2020). Accessed 12 Apr 2021
The Institute for Ethical AI in Education The Ethical Framework for AI in Education (IEAIED). https://fb77c667c4d6e21c1e06.b-cdn.net/wp-content/uploads/2021/03/The-Ethical-Framework-for-AI-in-Education-Institute-for-Ethical-AI-in-Education-Final-Report.pdf (2021). Accessed 12 Apr 2021
The Institute for Ethical AI in Education The Ethical Framework for AI in Education (n.d.). Available at: https://www.buckingham.ac.uk/wp-content/uploads/2021/03/The-Institute-for-Ethical-AI-in-Education-The-Ethical-Framework-for-AI-in-Education.pdf . Accessed 4 July 2021
Tisseron, S., Tordo, F., Baddoura, R.: Testing Empathy with Robots: a model in four dimensions and sixteen ítems. Int. J. Soc. Robot. 7 (1), 97–102 (2015)
UNESCO. Artificial intelligence in education. UNESCO. https://en.unesco.org/artificial-intelligence/education . (2019). Accessed 12 Apr 2021
Utterberg Modén, M., Tallvid, M., Lundin, J., Lindström, B.: Intelligent Tutoring Systems: Why Teachers Abandoned a Technology Aimed at Automating Teaching Processes. In: Proceedings of the 54th Hawaii International Conference on System Sciences, Maui, p. 1538 (2021)
van der Spoel, I., Noroozi, O., Schuurink, E., van Ginkel, S.: Teachers’ online teaching expectations and experiences during the Covid19-pandemic in the Netherlands. Eur. J. Teach. Educ. 43 (4), 623–638 (2020)
Weller, M.: Twenty years of EdTech. Educa. Rev. Online. 53 (4), 34–48 (2018)
Wenger, E.: Artificial intelligence and tutoring systems. Morgan Kauffman, Los Altos (1987)
World Economic Forum and The Boston Consulting Group. New vision for education unlocking the potential of technology industry agenda prepared in collaboration with the Boston consulting group. http://www3.weforum.org/docs/WEFUSA_NewVisionforEducation_Report2015.pdf (2015). Accessed 12 Apr 2021
Yampolskiy, R.V., Spellchecker, M.S.: Artificial intelligence safety and cybersecurity: a timeline of AI failures. arXiv:1610.07997 (2016)
Yu, H., Miao, C., Leung, C., White, T.J.: Towards AI-powered personalization in MOOC learning. Npj. Sci. Learn. 2 (1), 1–5 (2017)
Yu, H., Shen, Z., Miao, C., Leung, C., Lesser, V.R., Yang, Q.: Building ethics into artificial intelligence. arXiv:1812.02953 (2018)
Zemel, R., Wu Y., Swersky, K., Pitassi, T., Dwork, C.: Learning fair representations. In: International Conference on Machine Learning, pp. 325–333 (2013)
Zhang, Y., Liao, Q.V., Bellamy, R.K.E.: Effect of confidence and explanation on accuracy and trust calibration in AI-assisted decision making. In: Proceedings of the 2020 Conference on Fairness, Accountability, and Transparency. arXiv:2001.02114 (2020)
Zimmerman, B.J., Schunk, D.H.: Handbook of Self-Regulation of Learning and Performance. Routledge, Oxfordshire (2011)
Download references
Author information
Authors and affiliations.
Artificial Intelligence at University College, London, UK
Muhammad Ali Chaudhry
Department of Computer Science, University College, London, UK
You can also search for this author in PubMed Google Scholar
Corresponding author
Correspondence to Muhammad Ali Chaudhry .
Additional information
Publisher's note.
Springer Nature remains neutral with regard to jurisdictional claims in published maps and institutional affiliations.
Rights and permissions
Open Access This article is licensed under a Creative Commons Attribution 4.0 International License, which permits use, sharing, adaptation, distribution and reproduction in any medium or format, as long as you give appropriate credit to the original author(s) and the source, provide a link to the Creative Commons licence, and indicate if changes were made. The images or other third party material in this article are included in the article's Creative Commons licence, unless indicated otherwise in a credit line to the material. If material is not included in the article's Creative Commons licence and your intended use is not permitted by statutory regulation or exceeds the permitted use, you will need to obtain permission directly from the copyright holder. To view a copy of this licence, visit http://creativecommons.org/licenses/by/4.0/ .
Reprints and permissions
About this article
Chaudhry, M.A., Kazim, E. Artificial Intelligence in Education (AIEd): a high-level academic and industry note 2021. AI Ethics 2 , 157–165 (2022). https://doi.org/10.1007/s43681-021-00074-z
Download citation
Received : 25 April 2021
Accepted : 17 June 2021
Published : 07 July 2021
Issue Date : February 2022
DOI : https://doi.org/10.1007/s43681-021-00074-z
Share this article
Anyone you share the following link with will be able to read this content:
Sorry, a shareable link is not currently available for this article.
Provided by the Springer Nature SharedIt content-sharing initiative
- Machine learning
- Learning science
- Artificial Intelligence in Education (AIEd)
- Intelligent Tutoring Systems (ITS)
- Find a journal
- Publish with us
- Track your research

IMAGES
VIDEO
COMMENTS
Google Scholar provides a simple way to broadly search for scholarly literature. Search across a wide variety of disciplines and sources: articles, theses, books, abstracts and court opinions.
Educational technology businesses are continually attempting to create novel solutions to expand access to education for individuals who cannot obtain adequate educational facilities. Social media as a learning tool has come a long way. ... Google Scholar [5] C.I. Büyükbaykal. Communication technologies and education in the information age.
Amidst the global digital transformation of educational institutions, digital technology has emerged as a significant area of interest among scholars. Such technologies have played an instrumental ...
Reimagining the role of technology in education: 2017 national education technology plan update. Retrieved from https://tech.ed.gov/netp/ (open in a new window) . (Open in a new window) Google Scholar
Furthermore, we used an all-inclusive Google Scholar search to include relevant key terms or to include studies found in the reference list of the peer-reviewed papers, and other key studies and reports related to the concepts studied by professional and international bodies. ... Journal of Special Education Technology, 37(1), 74-81. https ...
The bibliometric data provided by Google Scholar Metrics is generated by Google Scholar, which is the most comprehensive and least biased scientific and academic data source currently available, as reported in Delgado and Repiso (2013). As a second inclusion criterion, we have determined that they be journals to which the analysts, authors of ...
The blending of traditional classroom and digital classroom becomes almost imminent. But it is also equally important to understand the inherent merits and demerits of digital classroom. Use of computer technology can enhance the quality of education, but technology alone cannot be sufficient.
Google Scholar [3] Arztmann, M., Hornstra, L., Jeuring, J., & Kester, L. (2022). Effects of games in STEM education: A meta-analysis on the moderating role of student background characteristics. ... Review of seven educational technology journals, 1970-2011 British Journal of Educational Technology 2014 45 2 202-230. Crossref. Google Scholar [58]
While the combination of distance learning and educational technology presents a timely solution combating adverse consequences of school disruptions, there is a real risk of channeling learning opportunities through already-existing forms of digital disparity, especially for marginalized populations. ... Google Scholar. Bonal X., González S ...
In the past few decades, technology has completely transformed the world around us. Indeed, experts believe that the next big digital transformation in how we live, communicate, work, trade and learn will be driven by Artificial Intelligence (AI) [83]. This paper presents a high-level industrial and academic overview of AI in Education (AIEd). It presents the focus of latest research in AIEd ...